Malaysia
2025-04-29 14:14
IndustryReinforcement learning (RL)
#AIImpactOnForex
Reinforcement learning (RL) offers a unique approach to algorithmic trading in Forex. Instead of being explicitly programmed with specific trading rules, RL agents learn optimal strategies through a process of trial and error within a simulated market environment. The agent takes actions (e.g., buying or selling currency pairs) and receives rewards or penalties based on the outcomes of those actions.
Over time, the RL agent learns to identify the actions that maximize its cumulative reward, effectively discovering profitable trading strategies without explicit human guidance. This allows for the development of adaptive trading systems that can adjust to changing market dynamics and potentially uncover novel strategies that human programmers might not have conceived. The iterative learning process enables the agent to refine its decision-making over time, leading to potentially sophisticated and robust trading algorithms.
Like 0
FX2496010620
Trader
Hot content
Industry
Event-A comment a day,Keep rewards worthy up to$27
Industry
Nigeria Event Giveaway-Win₦5000 Mobilephone Credit
Industry
Nigeria Event Giveaway-Win ₦2500 MobilePhoneCredit
Industry
South Africa Event-Come&Win 240ZAR Phone Credit
Industry
Nigeria Event-Discuss Forex&Win2500NGN PhoneCredit
Industry
[Nigeria Event]Discuss&win 2500 Naira Phone Credit
Forum category
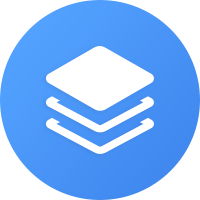
Platform
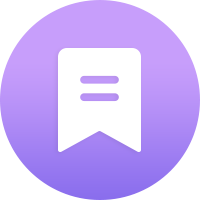
Exhibition
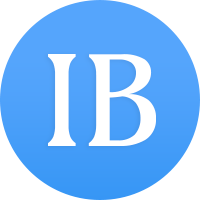
Agent
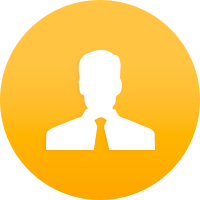
Recruitment
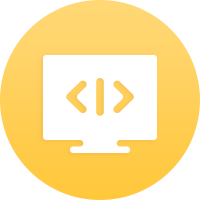
EA
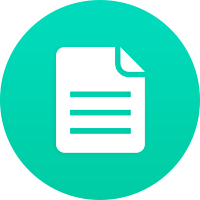
Industry
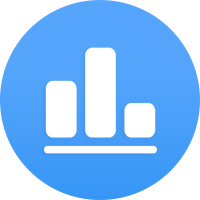
Market
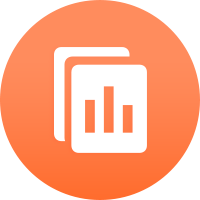
Index
Reinforcement learning (RL)
#AIImpactOnForex
Reinforcement learning (RL) offers a unique approach to algorithmic trading in Forex. Instead of being explicitly programmed with specific trading rules, RL agents learn optimal strategies through a process of trial and error within a simulated market environment. The agent takes actions (e.g., buying or selling currency pairs) and receives rewards or penalties based on the outcomes of those actions.
Over time, the RL agent learns to identify the actions that maximize its cumulative reward, effectively discovering profitable trading strategies without explicit human guidance. This allows for the development of adaptive trading systems that can adjust to changing market dynamics and potentially uncover novel strategies that human programmers might not have conceived. The iterative learning process enables the agent to refine its decision-making over time, leading to potentially sophisticated and robust trading algorithms.
Like 0
I want to comment, too
Submit
0Comments
There is no comment yet. Make the first one.
Submit
There is no comment yet. Make the first one.