Malaysia
2025-04-28 17:31
IndustryTuning in AI Models for Currency Forecasting
#AIImpactOnForex
Tuning AI models for currency forecasting involves optimizing machine learning algorithms to predict currency exchange rates. Key techniques include:
1. Feature Selection: Identifying relevant economic indicators, historical data, and market sentiment to improve model accuracy.
2. Model Selection: Using algorithms such as neural networks, decision trees, and support vector machines (SVM) that can capture complex patterns in financial data.
3. Hyperparameter Optimization: Adjusting parameters like learning rate, regularization strength, and network layers to enhance model performance.
4. Data Preprocessing: Handling missing data, scaling features, and removing noise to ensure the model works with high-quality input.
5. Evaluation Metrics: Using metrics like Mean Absolute Error (MAE) and Root Mean Squared Error (RMSE) to assess prediction accuracy.
6. Backtesting: Testing the model on historical data to evaluate its performance before live deployment.
Tuning requires a balance of computational resources, expert insights into financial markets, and iterative refinement to achieve reliable forecasting results.
Like 0
dondo6212
Trader
Hot content
Industry
Event-A comment a day,Keep rewards worthy up to$27
Industry
Nigeria Event Giveaway-Win₦5000 Mobilephone Credit
Industry
Nigeria Event Giveaway-Win ₦2500 MobilePhoneCredit
Industry
South Africa Event-Come&Win 240ZAR Phone Credit
Industry
Nigeria Event-Discuss Forex&Win2500NGN PhoneCredit
Industry
[Nigeria Event]Discuss&win 2500 Naira Phone Credit
Forum category
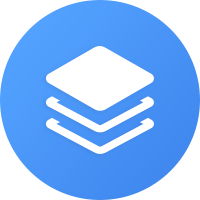
Platform
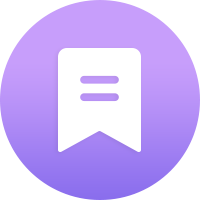
Exhibition
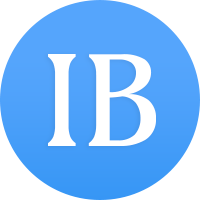
Agent
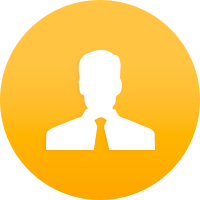
Recruitment
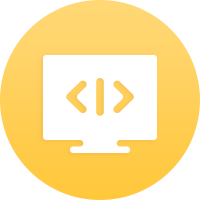
EA
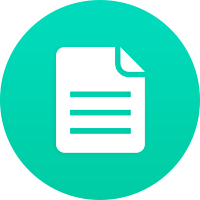
Industry
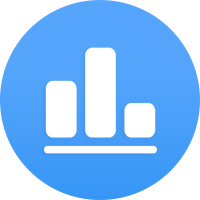
Market
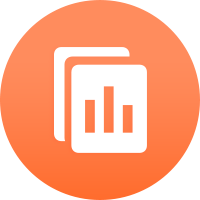
Index
Tuning in AI Models for Currency Forecasting
#AIImpactOnForex
Tuning AI models for currency forecasting involves optimizing machine learning algorithms to predict currency exchange rates. Key techniques include:
1. Feature Selection: Identifying relevant economic indicators, historical data, and market sentiment to improve model accuracy.
2. Model Selection: Using algorithms such as neural networks, decision trees, and support vector machines (SVM) that can capture complex patterns in financial data.
3. Hyperparameter Optimization: Adjusting parameters like learning rate, regularization strength, and network layers to enhance model performance.
4. Data Preprocessing: Handling missing data, scaling features, and removing noise to ensure the model works with high-quality input.
5. Evaluation Metrics: Using metrics like Mean Absolute Error (MAE) and Root Mean Squared Error (RMSE) to assess prediction accuracy.
6. Backtesting: Testing the model on historical data to evaluate its performance before live deployment.
Tuning requires a balance of computational resources, expert insights into financial markets, and iterative refinement to achieve reliable forecasting results.
Like 0
I want to comment, too
Submit
0Comments
There is no comment yet. Make the first one.
Submit
There is no comment yet. Make the first one.