Malaysia
2025-04-28 17:22
IndustryAI Transfer Learning for Forex Predictions
#AIImpactOnForex
AI transfer learning for forex (foreign exchange) predictions involves taking a pre-trained machine learning model, typically trained on a different but related task, and adapting it to make predictions on forex market data. The idea is to leverage the knowledge acquired by the model from one domain and apply it to another domain with limited data or less extensive training. This approach is particularly useful in forex predictions, where historical data can be sparse or noisy, and training a model from scratch might be resource-intensive.
In this context, a model pre-trained on financial data, stock market trends, or even other time-series forecasting tasks can be fine-tuned on specific forex data, such as currency pairs' price movements. By transferring the learned features or patterns, the model can perform better with fewer forex-specific data points, reducing training time and improving prediction accuracy. This method is especially effective when data availability is an issue, as it allows for the reuse of robust features learned in broader financial contexts.
Like 0
FX4285333292
Trader
Hot content
Industry
Event-A comment a day,Keep rewards worthy up to$27
Industry
Nigeria Event Giveaway-Win₦5000 Mobilephone Credit
Industry
Nigeria Event Giveaway-Win ₦2500 MobilePhoneCredit
Industry
South Africa Event-Come&Win 240ZAR Phone Credit
Industry
Nigeria Event-Discuss Forex&Win2500NGN PhoneCredit
Industry
[Nigeria Event]Discuss&win 2500 Naira Phone Credit
Forum category
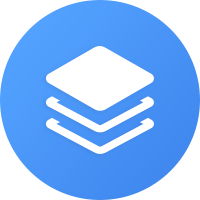
Platform
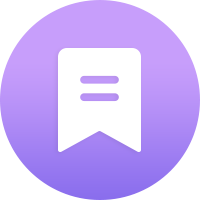
Exhibition
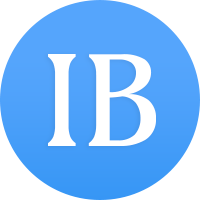
Agent
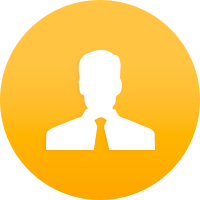
Recruitment
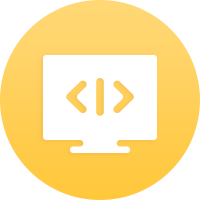
EA
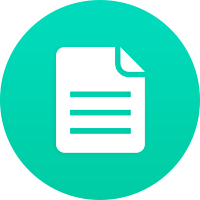
Industry
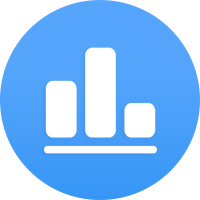
Market
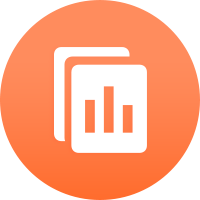
Index
AI Transfer Learning for Forex Predictions
#AIImpactOnForex
AI transfer learning for forex (foreign exchange) predictions involves taking a pre-trained machine learning model, typically trained on a different but related task, and adapting it to make predictions on forex market data. The idea is to leverage the knowledge acquired by the model from one domain and apply it to another domain with limited data or less extensive training. This approach is particularly useful in forex predictions, where historical data can be sparse or noisy, and training a model from scratch might be resource-intensive.
In this context, a model pre-trained on financial data, stock market trends, or even other time-series forecasting tasks can be fine-tuned on specific forex data, such as currency pairs' price movements. By transferring the learned features or patterns, the model can perform better with fewer forex-specific data points, reducing training time and improving prediction accuracy. This method is especially effective when data availability is an issue, as it allows for the reuse of robust features learned in broader financial contexts.
Like 0
I want to comment, too
Submit
0Comments
There is no comment yet. Make the first one.
Submit
There is no comment yet. Make the first one.