Vietnam
2025-04-28 16:28
IndustryReinforcement Learning in Currency Trading Models
#AIImpactOnForex
Reinforcement Learning in Currency Trading Models
Reinforcement learning (RL) is an AI approach where models learn to make trading decisions by interacting with the currency market environment and optimizing their strategies through trial and error. In currency trading, RL agents are trained to maximize cumulative returns by deciding when to buy, sell, or hold currencies based on market data. Techniques like Q-learning, Deep Q-Networks (DQN), and Proximal Policy Optimization (PPO) are commonly used. RL models can adapt to changing market dynamics without relying on pre-defined rules, making them highly flexible. However, they require careful training to handle market volatility and avoid overfitting to historical data.
Like 0
téo705
Trader
Hot content
Industry
Event-A comment a day,Keep rewards worthy up to$27
Industry
Nigeria Event Giveaway-Win₦5000 Mobilephone Credit
Industry
Nigeria Event Giveaway-Win ₦2500 MobilePhoneCredit
Industry
South Africa Event-Come&Win 240ZAR Phone Credit
Industry
Nigeria Event-Discuss Forex&Win2500NGN PhoneCredit
Industry
[Nigeria Event]Discuss&win 2500 Naira Phone Credit
Forum category
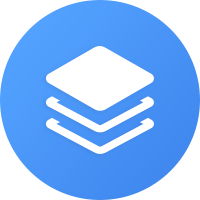
Platform
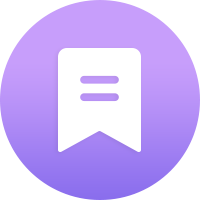
Exhibition
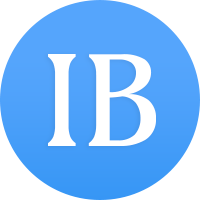
Agent
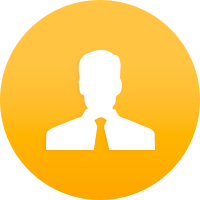
Recruitment
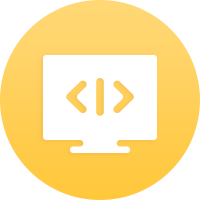
EA
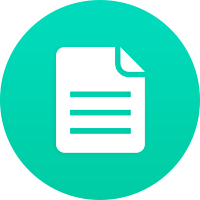
Industry
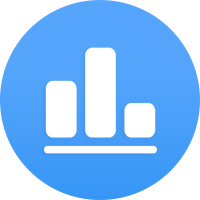
Market
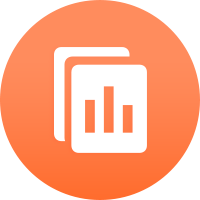
Index
Reinforcement Learning in Currency Trading Models
#AIImpactOnForex
Reinforcement Learning in Currency Trading Models
Reinforcement learning (RL) is an AI approach where models learn to make trading decisions by interacting with the currency market environment and optimizing their strategies through trial and error. In currency trading, RL agents are trained to maximize cumulative returns by deciding when to buy, sell, or hold currencies based on market data. Techniques like Q-learning, Deep Q-Networks (DQN), and Proximal Policy Optimization (PPO) are commonly used. RL models can adapt to changing market dynamics without relying on pre-defined rules, making them highly flexible. However, they require careful training to handle market volatility and avoid overfitting to historical data.
Like 0
I want to comment, too
Submit
0Comments
There is no comment yet. Make the first one.
Submit
There is no comment yet. Make the first one.