Thailand
2025-04-28 12:54
IndustryEnsemble LSTM and GRU modelsfor currency predictio
#CurrencyPairPrediction
Ensemble models combining Long Short-Term Memory (LSTM) networks and Gated Recurrent Units (GRUs) have emerged as a promising approach for enhancing the accuracy and robustness of Forex (FX) prediction. Both LSTM and GRU are types of Recurrent Neural Networks (RNNs) specifically designed to handle sequential data, making them well-suited for time series forecasting tasks like currency price prediction.
LSTMs excel at capturing long-term dependencies in the data through their sophisticated memory cell mechanism, which allows them to selectively retain or forget information over extended sequences. GRUs, while having a simpler architecture with fewer parameters, can also effectively model sequential dependencies and often exhibit comparable or even superior performance to LSTMs in certain scenarios, particularly when dealing with shorter sequences or limited data.
Ensembling LSTM and GRU models can leverage the distinct strengths of each architecture. One common approach involves training multiple LSTM and GRU networks with different initializations, architectures (e.g., number of layers, number of hidden units), or training data subsets. The predictions from these individual models are then combined using techniques like simple averaging, weighted averaging (where weights are determined based on validation performance), or more advanced ensemble learning methods like stacking.
The rationale behind ensembling is to reduce the risk of relying on a single model that might be susceptible to overfitting or might not capture all the underlying patterns in the complex FX market. By combining the diverse perspectives of multiple models, the ensemble can potentially smooth out individual model errors, improve generalization to unseen data, and provide more stable and accurate predictions.
Furthermore, hybrid ensemble architectures that integrate LSTM and GRU layers within the same network are also being explored. These models aim to allow the network to simultaneously learn both long-term and potentially shorter-term dependencies more effectively. The outputs from the LSTM and GRU components can be fused at various stages of the network to produce the final prediction.
However, it's important to note that while ensemble methods can often improve predictive performance, they also increase the complexity of the modeling process and require more computational resources for training and inference. Careful selection of the ensemble members, appropriate combination techniques, and rigorous evaluation are essential to realize the potential benefits of LSTM and GRU ensembles in Forex forecasting.
Like 0
kualar
Trader
Hot content
Industry
Event-A comment a day,Keep rewards worthy up to$27
Industry
Nigeria Event Giveaway-Win₦5000 Mobilephone Credit
Industry
Nigeria Event Giveaway-Win ₦2500 MobilePhoneCredit
Industry
South Africa Event-Come&Win 240ZAR Phone Credit
Industry
Nigeria Event-Discuss Forex&Win2500NGN PhoneCredit
Industry
[Nigeria Event]Discuss&win 2500 Naira Phone Credit
Forum category
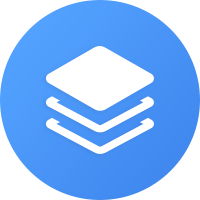
Platform
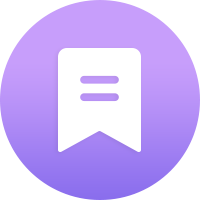
Exhibition
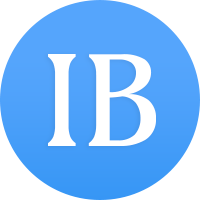
Agent
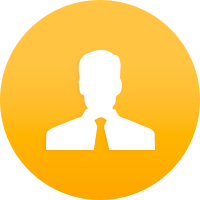
Recruitment
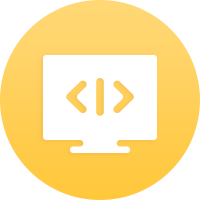
EA
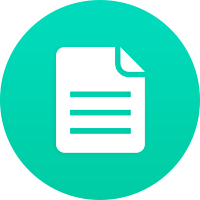
Industry
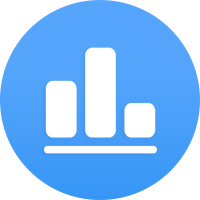
Market
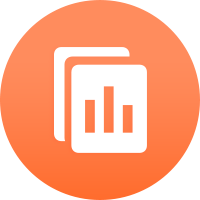
Index
Ensemble LSTM and GRU modelsfor currency predictio
#CurrencyPairPrediction
Ensemble models combining Long Short-Term Memory (LSTM) networks and Gated Recurrent Units (GRUs) have emerged as a promising approach for enhancing the accuracy and robustness of Forex (FX) prediction. Both LSTM and GRU are types of Recurrent Neural Networks (RNNs) specifically designed to handle sequential data, making them well-suited for time series forecasting tasks like currency price prediction.
LSTMs excel at capturing long-term dependencies in the data through their sophisticated memory cell mechanism, which allows them to selectively retain or forget information over extended sequences. GRUs, while having a simpler architecture with fewer parameters, can also effectively model sequential dependencies and often exhibit comparable or even superior performance to LSTMs in certain scenarios, particularly when dealing with shorter sequences or limited data.
Ensembling LSTM and GRU models can leverage the distinct strengths of each architecture. One common approach involves training multiple LSTM and GRU networks with different initializations, architectures (e.g., number of layers, number of hidden units), or training data subsets. The predictions from these individual models are then combined using techniques like simple averaging, weighted averaging (where weights are determined based on validation performance), or more advanced ensemble learning methods like stacking.
The rationale behind ensembling is to reduce the risk of relying on a single model that might be susceptible to overfitting or might not capture all the underlying patterns in the complex FX market. By combining the diverse perspectives of multiple models, the ensemble can potentially smooth out individual model errors, improve generalization to unseen data, and provide more stable and accurate predictions.
Furthermore, hybrid ensemble architectures that integrate LSTM and GRU layers within the same network are also being explored. These models aim to allow the network to simultaneously learn both long-term and potentially shorter-term dependencies more effectively. The outputs from the LSTM and GRU components can be fused at various stages of the network to produce the final prediction.
However, it's important to note that while ensemble methods can often improve predictive performance, they also increase the complexity of the modeling process and require more computational resources for training and inference. Careful selection of the ensemble members, appropriate combination techniques, and rigorous evaluation are essential to realize the potential benefits of LSTM and GRU ensembles in Forex forecasting.
Like 0
I want to comment, too
Submit
0Comments
There is no comment yet. Make the first one.
Submit
There is no comment yet. Make the first one.