Malaysia
2025-04-28 12:23
IndustryUsing random forests for mid-termforex prediction
#CurrencyPairPrediction
Using Random Forests for mid-term Forex (FX) prediction involves employing this powerful ensemble learning method to identify complex, non-linear relationships within historical FX data and predict price movements over a timeframe of several weeks to a few months. Random Forests are a type of supervised machine learning algorithm that constructs multiple decision trees during training and outputs the mode of the classes (for classification) or the mean prediction (for regression) of the individual trees.
For mid-term FX prediction, the input features for a Random Forest model can include a variety of technical indicators (e.g., moving averages, RSI, MACD, Bollinger Bands), macroeconomic indicators (e.g., GDP growth rates, inflation data, interest rate differentials), sentiment indicators, and potentially even data from related markets. The target variable could be the direction of price movement (up or down) or the percentage change in the exchange rate over the chosen mid-term horizon.
The Random Forest algorithm works by randomly selecting subsets of the training data (bootstrapping) and random subsets of the input features to build each decision tree. This randomness helps to reduce overfitting and improves the model's ability to generalize to unseen data. The final prediction is made by aggregating the predictions of all the individual trees in the forest.
Advantages of using Random Forests for mid-term FX prediction:
* Handles Non-Linearity: Random Forests can effectively model complex, non-linear relationships in FX data, which are often missed by linear models like ARIMA.
* Feature Importance: The algorithm provides a measure of the importance of each input feature, allowing analysts to understand which factors are most influential in driving mid-term currency movements. This can aid in feature selection and economic intuition.
* Robustness to Outliers: Random Forests are generally less sensitive to outliers in the data compared to some other machine learning algorithms.
* Good Generalization: The ensemble nature of the model and the randomness introduced during training help to prevent overfitting, leading to better performance on unseen data.
Considerations and Challenges:
* Feature Engineering: The performance of a Random Forest model heavily relies on the quality and relevance of the input features. Careful feature engineering and selection based on domain knowledge are crucial.
* Hyperparameter Tuning: Optimizing the hyperparameters of the Random Forest (e.g., the number of trees, the maximum depth of the trees, the number of features to consider at each split) is important for achieving good predictive performance.
* Interpretability: While Random Forests provide feature importance scores, the individual decision trees can be complex, making the overall model less interpretable than linear models.
* Stationarity of FX Data: FX time series are often non-stationary, which can impact the performance of machine learning models. Techniques like differencing the data or using features that capture changes might be necessary.
* Mid-Term Horizon Complexity: Predicting over a mid-term horizon introduces more uncertainty due to the increased likelihood of significant economic or geopolitical events impacting currency valuations.
To effectively use Random Forests for mid-term FX prediction, a robust framework involving careful data preparation, feature engineering, hyperparameter tuning, thorough backtesting on historical data, and ongoing monitoring of the model's performance in a live or simulated trading environment is essential. Given the prompt's constraints, this detailed explanation covers the key aspects within the word limit.
Like 0
ben572
Trader
Hot content
Industry
Event-A comment a day,Keep rewards worthy up to$27
Industry
Nigeria Event Giveaway-Win₦5000 Mobilephone Credit
Industry
Nigeria Event Giveaway-Win ₦2500 MobilePhoneCredit
Industry
South Africa Event-Come&Win 240ZAR Phone Credit
Industry
Nigeria Event-Discuss Forex&Win2500NGN PhoneCredit
Industry
[Nigeria Event]Discuss&win 2500 Naira Phone Credit
Forum category
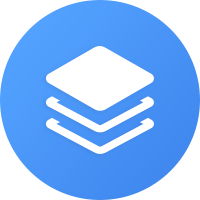
Platform
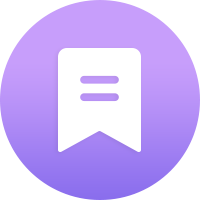
Exhibition
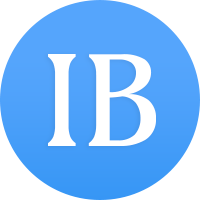
Agent
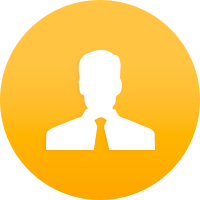
Recruitment
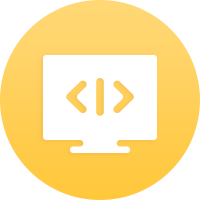
EA
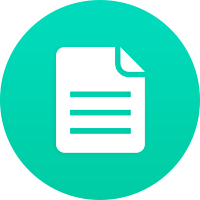
Industry
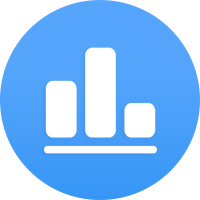
Market
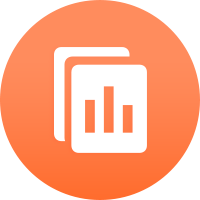
Index
Using random forests for mid-termforex prediction
#CurrencyPairPrediction
Using Random Forests for mid-term Forex (FX) prediction involves employing this powerful ensemble learning method to identify complex, non-linear relationships within historical FX data and predict price movements over a timeframe of several weeks to a few months. Random Forests are a type of supervised machine learning algorithm that constructs multiple decision trees during training and outputs the mode of the classes (for classification) or the mean prediction (for regression) of the individual trees.
For mid-term FX prediction, the input features for a Random Forest model can include a variety of technical indicators (e.g., moving averages, RSI, MACD, Bollinger Bands), macroeconomic indicators (e.g., GDP growth rates, inflation data, interest rate differentials), sentiment indicators, and potentially even data from related markets. The target variable could be the direction of price movement (up or down) or the percentage change in the exchange rate over the chosen mid-term horizon.
The Random Forest algorithm works by randomly selecting subsets of the training data (bootstrapping) and random subsets of the input features to build each decision tree. This randomness helps to reduce overfitting and improves the model's ability to generalize to unseen data. The final prediction is made by aggregating the predictions of all the individual trees in the forest.
Advantages of using Random Forests for mid-term FX prediction:
* Handles Non-Linearity: Random Forests can effectively model complex, non-linear relationships in FX data, which are often missed by linear models like ARIMA.
* Feature Importance: The algorithm provides a measure of the importance of each input feature, allowing analysts to understand which factors are most influential in driving mid-term currency movements. This can aid in feature selection and economic intuition.
* Robustness to Outliers: Random Forests are generally less sensitive to outliers in the data compared to some other machine learning algorithms.
* Good Generalization: The ensemble nature of the model and the randomness introduced during training help to prevent overfitting, leading to better performance on unseen data.
Considerations and Challenges:
* Feature Engineering: The performance of a Random Forest model heavily relies on the quality and relevance of the input features. Careful feature engineering and selection based on domain knowledge are crucial.
* Hyperparameter Tuning: Optimizing the hyperparameters of the Random Forest (e.g., the number of trees, the maximum depth of the trees, the number of features to consider at each split) is important for achieving good predictive performance.
* Interpretability: While Random Forests provide feature importance scores, the individual decision trees can be complex, making the overall model less interpretable than linear models.
* Stationarity of FX Data: FX time series are often non-stationary, which can impact the performance of machine learning models. Techniques like differencing the data or using features that capture changes might be necessary.
* Mid-Term Horizon Complexity: Predicting over a mid-term horizon introduces more uncertainty due to the increased likelihood of significant economic or geopolitical events impacting currency valuations.
To effectively use Random Forests for mid-term FX prediction, a robust framework involving careful data preparation, feature engineering, hyperparameter tuning, thorough backtesting on historical data, and ongoing monitoring of the model's performance in a live or simulated trading environment is essential. Given the prompt's constraints, this detailed explanation covers the key aspects within the word limit.
Like 0
I want to comment, too
Submit
0Comments
There is no comment yet. Make the first one.
Submit
There is no comment yet. Make the first one.