Malaysia
2025-04-28 12:13
IndustryPredicting forex using unsupervisedpretraining
#CurrencyPairPrediction
Predicting Forex (FX) movements using unsupervised pretraining involves leveraging large amounts of unlabeled FX market data to learn general representations and patterns, which can then be fine-tuned for specific prediction tasks. This approach has gained traction in various fields, including natural language processing and computer vision, where pretraining on massive datasets helps models learn useful features before being applied to downstream tasks with smaller labeled datasets.
In the context of FX, unsupervised pretraining could involve training deep learning models, such as autoencoders or transformer networks, on vast quantities of historical price data, order book information, or even financial news text. The goal during pretraining is not to predict a specific target variable (like future price movements) but rather to learn meaningful representations of the input data. For example, an autoencoder might be trained to encode the input data into a lower-dimensional representation and then decode it back to the original input, forcing the model to learn salient features. Transformer networks can be pretrained using tasks like masked language modeling on financial news or predicting future time steps in a sequence of price data.
Once the pretraining phase is complete, the learned representations or the pretrained model's weights can be used as a starting point for a supervised learning task, such as predicting the direction of price movement or volatility. This involves adding a task-specific layer (e.g., a classification or regression layer) on top of the pretrained model and then fine-tuning the entire architecture on a smaller labeled dataset of FX prices and corresponding target variables.
The potential benefits of unsupervised pretraining in FX prediction include:
* Improved Performance with Limited Labeled Data: FX datasets can be large, but the truly informative labeled data for specific prediction tasks might be relatively scarce. Pretraining on massive unlabeled data can help the model learn general FX market dynamics, leading to better performance even with limited labeled data for fine-tuning.
* Learning Robust Feature Representations: Unsupervised pretraining can enable the model to automatically learn complex and potentially more robust feature representations from the raw data compared to relying solely on hand-engineered features or training from scratch on a smaller labeled dataset.
* Better Generalization: The features learned during pretraining on a large and diverse dataset might help the model generalize better to unseen market conditions and reduce overfitting on the labeled data.
However, there are also challenges associated with this approach in FX prediction. The signal-to-noise ratio in FX data can be low, and identifying truly meaningful patterns through unsupervised learning can be difficult. The choice of pretraining task and model architecture needs to be carefully considered for the specific characteristics of FX data. Additionally, the effectiveness of pretraining often depends on the size and quality of the unlabeled data used.
Like 0
laroy
Trader
Hot content
Industry
Event-A comment a day,Keep rewards worthy up to$27
Industry
Nigeria Event Giveaway-Win₦5000 Mobilephone Credit
Industry
Nigeria Event Giveaway-Win ₦2500 MobilePhoneCredit
Industry
South Africa Event-Come&Win 240ZAR Phone Credit
Industry
Nigeria Event-Discuss Forex&Win2500NGN PhoneCredit
Industry
[Nigeria Event]Discuss&win 2500 Naira Phone Credit
Forum category
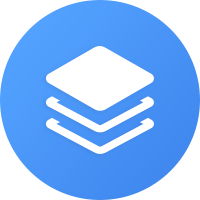
Platform
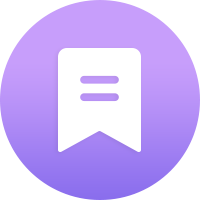
Exhibition
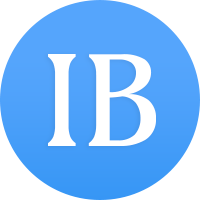
Agent
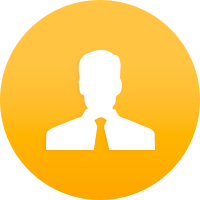
Recruitment
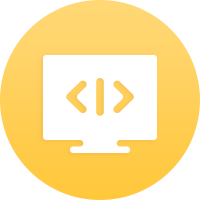
EA
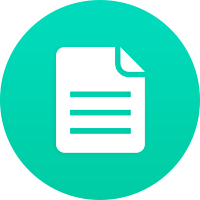
Industry
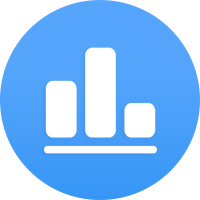
Market
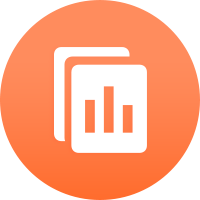
Index
Predicting forex using unsupervisedpretraining
#CurrencyPairPrediction
Predicting Forex (FX) movements using unsupervised pretraining involves leveraging large amounts of unlabeled FX market data to learn general representations and patterns, which can then be fine-tuned for specific prediction tasks. This approach has gained traction in various fields, including natural language processing and computer vision, where pretraining on massive datasets helps models learn useful features before being applied to downstream tasks with smaller labeled datasets.
In the context of FX, unsupervised pretraining could involve training deep learning models, such as autoencoders or transformer networks, on vast quantities of historical price data, order book information, or even financial news text. The goal during pretraining is not to predict a specific target variable (like future price movements) but rather to learn meaningful representations of the input data. For example, an autoencoder might be trained to encode the input data into a lower-dimensional representation and then decode it back to the original input, forcing the model to learn salient features. Transformer networks can be pretrained using tasks like masked language modeling on financial news or predicting future time steps in a sequence of price data.
Once the pretraining phase is complete, the learned representations or the pretrained model's weights can be used as a starting point for a supervised learning task, such as predicting the direction of price movement or volatility. This involves adding a task-specific layer (e.g., a classification or regression layer) on top of the pretrained model and then fine-tuning the entire architecture on a smaller labeled dataset of FX prices and corresponding target variables.
The potential benefits of unsupervised pretraining in FX prediction include:
* Improved Performance with Limited Labeled Data: FX datasets can be large, but the truly informative labeled data for specific prediction tasks might be relatively scarce. Pretraining on massive unlabeled data can help the model learn general FX market dynamics, leading to better performance even with limited labeled data for fine-tuning.
* Learning Robust Feature Representations: Unsupervised pretraining can enable the model to automatically learn complex and potentially more robust feature representations from the raw data compared to relying solely on hand-engineered features or training from scratch on a smaller labeled dataset.
* Better Generalization: The features learned during pretraining on a large and diverse dataset might help the model generalize better to unseen market conditions and reduce overfitting on the labeled data.
However, there are also challenges associated with this approach in FX prediction. The signal-to-noise ratio in FX data can be low, and identifying truly meaningful patterns through unsupervised learning can be difficult. The choice of pretraining task and model architecture needs to be carefully considered for the specific characteristics of FX data. Additionally, the effectiveness of pretraining often depends on the size and quality of the unlabeled data used.
Like 0
I want to comment, too
Submit
0Comments
There is no comment yet. Make the first one.
Submit
There is no comment yet. Make the first one.