Malaysia
2025-04-28 12:04
IndustryFX prediction using hierarchicaltime series models
#CurrencyPairPrediction
Predicting Forex (FX) movements using hierarchical time series (HTS) models involves structuring the currency market data into a hierarchy and then forecasting at different levels of this hierarchy to improve prediction accuracy and consistency. In the context of FX, a hierarchy could be based on various groupings, such as major pairs, minor pairs, exotic pairs, or classifications based on geographical regions or economic relationships.
The fundamental idea behind HTS models is that forecasting at a more aggregated level (e.g., forecasting the average movement of all major currency pairs) can often be more stable and predictable than forecasting individual series due to the smoothing effect of aggregation. These top-level forecasts can then be disaggregated down to the individual currency pair level, often incorporating information from the bottom-level series themselves. This reconciliation process ensures that the forecasts across all levels of the hierarchy are consistent and add up appropriately.
Several statistical and machine learning techniques can be adapted for hierarchical forecasting. Traditional time series methods like ARIMA or Exponential Smoothing can be applied at each level of the hierarchy. More advanced approaches involve specialized HTS models that explicitly account for the hierarchical structure during the forecasting process.
In the context of FX, a simple hierarchy could have a top level representing a global FX index (if one is constructed), an intermediate level for major currency groups (e.g., G7 currencies), and the bottom level for individual currency pairs (e.g., EUR/USD, GBP/JPY). By forecasting the overall trend at the top level and then distributing this forecast down to the individual pairs based on their historical relationships and individual forecasts, HTS models can potentially capture both the broad market movements and the specific dynamics of each pair.
One potential advantage of using HTS models in FX prediction is the ability to leverage information across related currency pairs. For instance, strong movements in one major currency might provide a signal for related minor or exotic pairs. The hierarchical structure can also help in identifying inconsistencies or outliers in individual pair forecasts when compared to the aggregated levels. Furthermore, HTS models can handle missing data or noisy signals at the individual pair level by borrowing strength from the more stable aggregate forecasts. However, constructing an appropriate and meaningful hierarchy for FX markets and choosing the right aggregation and disaggregation methods are crucial for the model's performance. The complexity of FX relationships might also require more sophisticated hierarchical structures and modeling techniques.
Like 0
uzi352
Trader
Hot content
Industry
Event-A comment a day,Keep rewards worthy up to$27
Industry
Nigeria Event Giveaway-Win₦5000 Mobilephone Credit
Industry
Nigeria Event Giveaway-Win ₦2500 MobilePhoneCredit
Industry
South Africa Event-Come&Win 240ZAR Phone Credit
Industry
Nigeria Event-Discuss Forex&Win2500NGN PhoneCredit
Industry
[Nigeria Event]Discuss&win 2500 Naira Phone Credit
Forum category
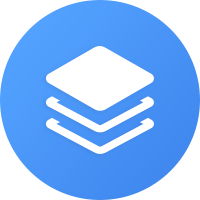
Platform
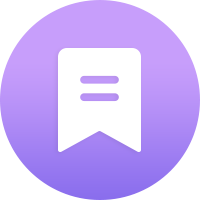
Exhibition
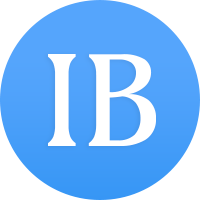
Agent
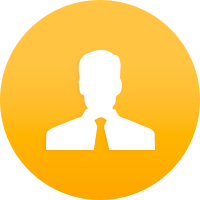
Recruitment
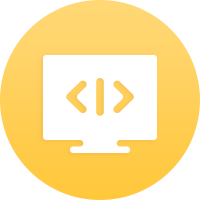
EA
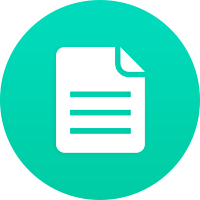
Industry
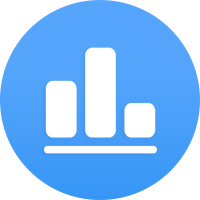
Market
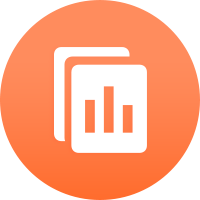
Index
FX prediction using hierarchicaltime series models
#CurrencyPairPrediction
Predicting Forex (FX) movements using hierarchical time series (HTS) models involves structuring the currency market data into a hierarchy and then forecasting at different levels of this hierarchy to improve prediction accuracy and consistency. In the context of FX, a hierarchy could be based on various groupings, such as major pairs, minor pairs, exotic pairs, or classifications based on geographical regions or economic relationships.
The fundamental idea behind HTS models is that forecasting at a more aggregated level (e.g., forecasting the average movement of all major currency pairs) can often be more stable and predictable than forecasting individual series due to the smoothing effect of aggregation. These top-level forecasts can then be disaggregated down to the individual currency pair level, often incorporating information from the bottom-level series themselves. This reconciliation process ensures that the forecasts across all levels of the hierarchy are consistent and add up appropriately.
Several statistical and machine learning techniques can be adapted for hierarchical forecasting. Traditional time series methods like ARIMA or Exponential Smoothing can be applied at each level of the hierarchy. More advanced approaches involve specialized HTS models that explicitly account for the hierarchical structure during the forecasting process.
In the context of FX, a simple hierarchy could have a top level representing a global FX index (if one is constructed), an intermediate level for major currency groups (e.g., G7 currencies), and the bottom level for individual currency pairs (e.g., EUR/USD, GBP/JPY). By forecasting the overall trend at the top level and then distributing this forecast down to the individual pairs based on their historical relationships and individual forecasts, HTS models can potentially capture both the broad market movements and the specific dynamics of each pair.
One potential advantage of using HTS models in FX prediction is the ability to leverage information across related currency pairs. For instance, strong movements in one major currency might provide a signal for related minor or exotic pairs. The hierarchical structure can also help in identifying inconsistencies or outliers in individual pair forecasts when compared to the aggregated levels. Furthermore, HTS models can handle missing data or noisy signals at the individual pair level by borrowing strength from the more stable aggregate forecasts. However, constructing an appropriate and meaningful hierarchy for FX markets and choosing the right aggregation and disaggregation methods are crucial for the model's performance. The complexity of FX relationships might also require more sophisticated hierarchical structures and modeling techniques.
Like 0
I want to comment, too
Submit
0Comments
There is no comment yet. Make the first one.
Submit
There is no comment yet. Make the first one.