Malaysia
2025-04-28 11:50
IndustryUsing Markov chains for forex priceprediction
#CurrencyPairPrediction
Using Markov chains for Forex (FX) price prediction involves modeling currency price movements as a sequence of states, where the probability of transitioning to a future state depends only on the current state. This aligns with the Markov property, which assumes the process is "memoryless."
To apply Markov chains, the continuous price movements of a currency pair are first discretized into a finite number of states. These states could represent price levels, percentage changes (e.g., up by >1%, stable, down by <1%), or defined market regimes (e.g., bull, bear, sideways). Historical price data is then used to estimate the transition probabilities between these states, forming a transition matrix. This matrix shows the likelihood of the price moving from one state to another within a given time step.
Once the transition matrix is established, it can be used to predict the probability of the currency pair being in a particular state at some point in the future. By multiplying the current state probability vector by the transition matrix repeatedly, one can forecast the long-term distribution of states.
Markov chains offer simplicity and can capture some short-term momentum or tendencies in price movements. They are relatively easy to implement and interpret. However, their effectiveness in Forex prediction is debated due to several limitations. The assumption of the Markov property might not strictly hold, as past price history beyond the immediate state could influence future movements. Financial markets are complex and influenced by numerous factors beyond just past price action, such as economic news, central bank policies, and global events, which are not inherently captured in a basic Markov chain model.
More sophisticated approaches involve Hidden Markov Models (HMMs), which introduce hidden states that are not directly observable but influence the observed price movements. These models can better capture underlying market regimes. Hybrid models combining Markov chains with other techniques like fuzzy time series have also been explored to improve forecasting accuracy. However, the inherent randomness and volatility of Forex markets make consistent and accurate prediction challenging for any single model, including those based on Markov chains.
Like 0
malay8643
Trader
Hot content
Industry
Event-A comment a day,Keep rewards worthy up to$27
Industry
Nigeria Event Giveaway-Win₦5000 Mobilephone Credit
Industry
Nigeria Event Giveaway-Win ₦2500 MobilePhoneCredit
Industry
South Africa Event-Come&Win 240ZAR Phone Credit
Industry
Nigeria Event-Discuss Forex&Win2500NGN PhoneCredit
Industry
[Nigeria Event]Discuss&win 2500 Naira Phone Credit
Forum category
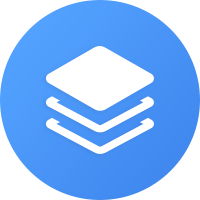
Platform
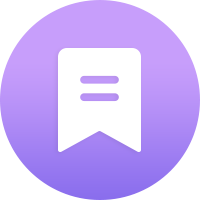
Exhibition
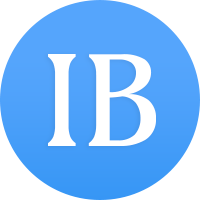
Agent
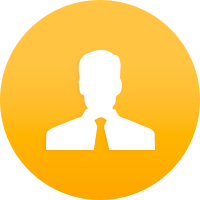
Recruitment
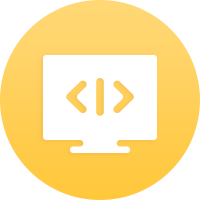
EA
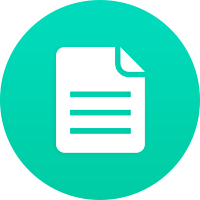
Industry
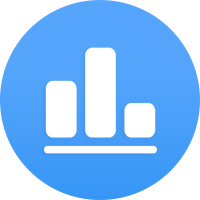
Market
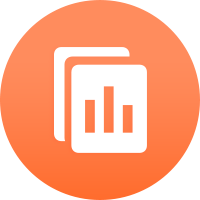
Index
Using Markov chains for forex priceprediction
#CurrencyPairPrediction
Using Markov chains for Forex (FX) price prediction involves modeling currency price movements as a sequence of states, where the probability of transitioning to a future state depends only on the current state. This aligns with the Markov property, which assumes the process is "memoryless."
To apply Markov chains, the continuous price movements of a currency pair are first discretized into a finite number of states. These states could represent price levels, percentage changes (e.g., up by >1%, stable, down by <1%), or defined market regimes (e.g., bull, bear, sideways). Historical price data is then used to estimate the transition probabilities between these states, forming a transition matrix. This matrix shows the likelihood of the price moving from one state to another within a given time step.
Once the transition matrix is established, it can be used to predict the probability of the currency pair being in a particular state at some point in the future. By multiplying the current state probability vector by the transition matrix repeatedly, one can forecast the long-term distribution of states.
Markov chains offer simplicity and can capture some short-term momentum or tendencies in price movements. They are relatively easy to implement and interpret. However, their effectiveness in Forex prediction is debated due to several limitations. The assumption of the Markov property might not strictly hold, as past price history beyond the immediate state could influence future movements. Financial markets are complex and influenced by numerous factors beyond just past price action, such as economic news, central bank policies, and global events, which are not inherently captured in a basic Markov chain model.
More sophisticated approaches involve Hidden Markov Models (HMMs), which introduce hidden states that are not directly observable but influence the observed price movements. These models can better capture underlying market regimes. Hybrid models combining Markov chains with other techniques like fuzzy time series have also been explored to improve forecasting accuracy. However, the inherent randomness and volatility of Forex markets make consistent and accurate prediction challenging for any single model, including those based on Markov chains.
Like 0
I want to comment, too
Submit
0Comments
There is no comment yet. Make the first one.
Submit
There is no comment yet. Make the first one.