Malaysia
2025-04-28 11:45
IndustryReal-time forex news clustering forprediction
#CurrencyPairPrediction
Real-time Forex (FX) news clustering for prediction involves using natural language processing (NLP) techniques to automatically group similar news articles related to currency movements as they are published. The goal is to identify key themes and prevailing sentiment that could influence exchange rates, providing traders with timely insights for their forecasting models.
The process typically begins with collecting a continuous stream of news articles from various financial news sources. NLP techniques are then applied to process this textual data. Sentiment analysis is a crucial step, aiming to determine the emotional tone (positive, negative, or neutral) expressed in each article towards specific currencies or economic factors. Topic modeling algorithms, such as Latent Dirichlet Allocation (LDA) or hierarchical clustering, can then group articles based on the similarity of their content, identifying the dominant themes and narratives driving market sentiment.
Real-time clustering allows for the dynamic identification of emerging news trends and their potential impact on currency pairs. For instance, a sudden cluster of negative news regarding a country's economic outlook could signal potential currency depreciation. Conversely, a cluster of positive reports about strong economic data might indicate a strengthening currency. The intensity and consistency of sentiment within a cluster can also provide clues about the potential magnitude and duration of the expected currency movement.
These clustered news items and their associated sentiment scores can then be integrated as features into Forex prediction models, potentially enhancing their accuracy. Machine learning models, including deep learning architectures like LSTMs or Transformers, can learn to identify the relationship between news clusters, sentiment, and subsequent currency price movements. By reacting to real-time news flow and sentiment, these models can adapt more quickly to market-moving events compared to models relying solely on historical price data. However, challenges remain in accurately capturing the nuanced relationships between news, sentiment, and currency movements, as well as dealing with noisy or contradictory information.
Like 0
lusy2642
Trader
Hot content
Industry
Event-A comment a day,Keep rewards worthy up to$27
Industry
Nigeria Event Giveaway-Win₦5000 Mobilephone Credit
Industry
Nigeria Event Giveaway-Win ₦2500 MobilePhoneCredit
Industry
South Africa Event-Come&Win 240ZAR Phone Credit
Industry
Nigeria Event-Discuss Forex&Win2500NGN PhoneCredit
Industry
[Nigeria Event]Discuss&win 2500 Naira Phone Credit
Forum category
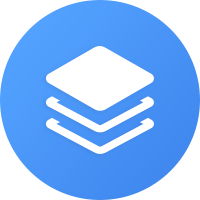
Platform
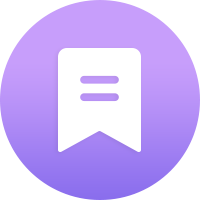
Exhibition
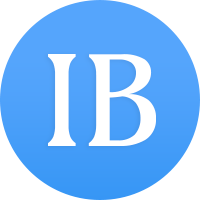
Agent
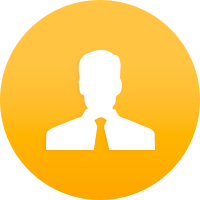
Recruitment
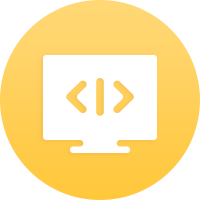
EA
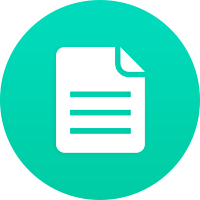
Industry
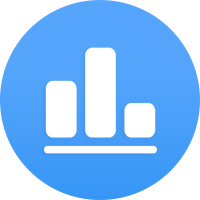
Market
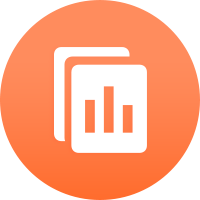
Index
Real-time forex news clustering forprediction
#CurrencyPairPrediction
Real-time Forex (FX) news clustering for prediction involves using natural language processing (NLP) techniques to automatically group similar news articles related to currency movements as they are published. The goal is to identify key themes and prevailing sentiment that could influence exchange rates, providing traders with timely insights for their forecasting models.
The process typically begins with collecting a continuous stream of news articles from various financial news sources. NLP techniques are then applied to process this textual data. Sentiment analysis is a crucial step, aiming to determine the emotional tone (positive, negative, or neutral) expressed in each article towards specific currencies or economic factors. Topic modeling algorithms, such as Latent Dirichlet Allocation (LDA) or hierarchical clustering, can then group articles based on the similarity of their content, identifying the dominant themes and narratives driving market sentiment.
Real-time clustering allows for the dynamic identification of emerging news trends and their potential impact on currency pairs. For instance, a sudden cluster of negative news regarding a country's economic outlook could signal potential currency depreciation. Conversely, a cluster of positive reports about strong economic data might indicate a strengthening currency. The intensity and consistency of sentiment within a cluster can also provide clues about the potential magnitude and duration of the expected currency movement.
These clustered news items and their associated sentiment scores can then be integrated as features into Forex prediction models, potentially enhancing their accuracy. Machine learning models, including deep learning architectures like LSTMs or Transformers, can learn to identify the relationship between news clusters, sentiment, and subsequent currency price movements. By reacting to real-time news flow and sentiment, these models can adapt more quickly to market-moving events compared to models relying solely on historical price data. However, challenges remain in accurately capturing the nuanced relationships between news, sentiment, and currency movements, as well as dealing with noisy or contradictory information.
Like 0
I want to comment, too
Submit
0Comments
There is no comment yet. Make the first one.
Submit
There is no comment yet. Make the first one.