Malaysia
2025-04-28 11:42
IndustryHybrid ARIMA-LSTM models forforex forecasting
#CurrencyPairPrediction
Hybrid ARIMA-LSTM models for Forex (FX) forecasting represent an innovative approach that combines the strengths of traditional linear time series models (ARIMA) with the non-linear pattern recognition capabilities of deep learning models (LSTM). This hybridization aims to capture both the linear and non-linear dependencies inherent in FX market data, potentially leading to more accurate and robust predictions than either model used in isolation.
The Autoregressive Integrated Moving Average (ARIMA) model excels at capturing linear relationships and short-term dependencies in time series data, such as autocorrelation and stationarity. It works by analyzing past values and forecast errors to predict future values based on linear combinations. However, ARIMA models are limited in their ability to model complex non-linear patterns that are often present in financial markets.
Long Short-Term Memory (LSTM) networks, a type of Recurrent Neural Network (RNN), are well-suited for learning long-term dependencies and capturing non-linear relationships in sequential data. They can automatically learn intricate patterns from large datasets without the need for explicit feature engineering. However, LSTMs may sometimes struggle to model the linear components of a time series as effectively as ARIMA.
In a hybrid ARIMA-LSTM model, the typical approach involves first decomposing the time series data into its linear and non-linear components. The ARIMA model is then applied to the linear part to capture its dynamics, and the LSTM network is trained on the residuals (the part of the data not explained by the ARIMA model) to learn the underlying non-linear patterns. The final forecast is obtained by combining the predictions from both models.
This hybrid approach can potentially leverage the strengths of both methodologies. ARIMA handles the linear dependencies and stationarity, while LSTM captures the complex non-linear relationships and long-term dependencies. By addressing both linear and non-linear aspects of FX market behavior, hybrid ARIMA-LSTM models have shown promise in achieving improved forecasting accuracy compared to using ARIMA or LSTM alone in various studies. However, the successful implementation of such models requires careful selection of model parameters, appropriate data preprocessing, and rigorous evaluation to ensure robust performance and avoid overfitting. The complexity of these models also means they can be more computationally intensive to train and optimize.
Like 0
atla
Trader
Hot content
Industry
Event-A comment a day,Keep rewards worthy up to$27
Industry
Nigeria Event Giveaway-Win₦5000 Mobilephone Credit
Industry
Nigeria Event Giveaway-Win ₦2500 MobilePhoneCredit
Industry
South Africa Event-Come&Win 240ZAR Phone Credit
Industry
Nigeria Event-Discuss Forex&Win2500NGN PhoneCredit
Industry
[Nigeria Event]Discuss&win 2500 Naira Phone Credit
Forum category
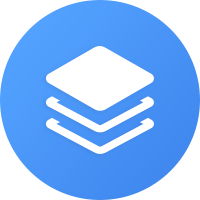
Platform
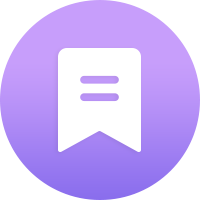
Exhibition
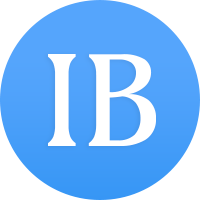
Agent
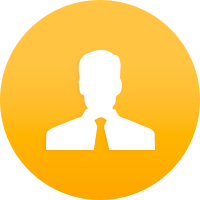
Recruitment
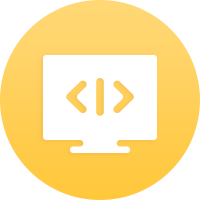
EA
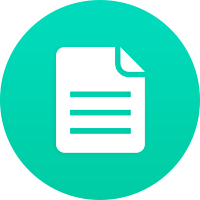
Industry
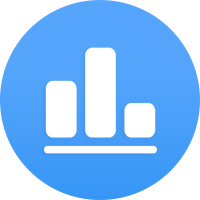
Market
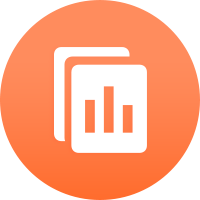
Index
Hybrid ARIMA-LSTM models forforex forecasting
#CurrencyPairPrediction
Hybrid ARIMA-LSTM models for Forex (FX) forecasting represent an innovative approach that combines the strengths of traditional linear time series models (ARIMA) with the non-linear pattern recognition capabilities of deep learning models (LSTM). This hybridization aims to capture both the linear and non-linear dependencies inherent in FX market data, potentially leading to more accurate and robust predictions than either model used in isolation.
The Autoregressive Integrated Moving Average (ARIMA) model excels at capturing linear relationships and short-term dependencies in time series data, such as autocorrelation and stationarity. It works by analyzing past values and forecast errors to predict future values based on linear combinations. However, ARIMA models are limited in their ability to model complex non-linear patterns that are often present in financial markets.
Long Short-Term Memory (LSTM) networks, a type of Recurrent Neural Network (RNN), are well-suited for learning long-term dependencies and capturing non-linear relationships in sequential data. They can automatically learn intricate patterns from large datasets without the need for explicit feature engineering. However, LSTMs may sometimes struggle to model the linear components of a time series as effectively as ARIMA.
In a hybrid ARIMA-LSTM model, the typical approach involves first decomposing the time series data into its linear and non-linear components. The ARIMA model is then applied to the linear part to capture its dynamics, and the LSTM network is trained on the residuals (the part of the data not explained by the ARIMA model) to learn the underlying non-linear patterns. The final forecast is obtained by combining the predictions from both models.
This hybrid approach can potentially leverage the strengths of both methodologies. ARIMA handles the linear dependencies and stationarity, while LSTM captures the complex non-linear relationships and long-term dependencies. By addressing both linear and non-linear aspects of FX market behavior, hybrid ARIMA-LSTM models have shown promise in achieving improved forecasting accuracy compared to using ARIMA or LSTM alone in various studies. However, the successful implementation of such models requires careful selection of model parameters, appropriate data preprocessing, and rigorous evaluation to ensure robust performance and avoid overfitting. The complexity of these models also means they can be more computationally intensive to train and optimize.
Like 0
I want to comment, too
Submit
0Comments
There is no comment yet. Make the first one.
Submit
There is no comment yet. Make the first one.