Malaysia
2025-04-28 11:32
IndustryPredicting forex pairs withreinforcement learning
#CurrencyPairPrediction
Predicting Forex (FX) pair movements using reinforcement learning (RL) agents involves training autonomous agents to learn optimal trading strategies through trial and error in a simulated FX market environment. These agents interact with the market, make trading decisions (buy, sell, or hold), and receive rewards (positive or negative) based on the profitability of their actions. Over time, the RL agent learns to maximize its cumulative reward, effectively discovering profitable trading strategies without explicit programming of specific rules.
The process typically involves defining a state space that includes relevant market data, such as historical price action, technical indicators (e.g., moving averages, RSI, MACD), order book information, and potentially even sentiment data. The agent then takes actions within an action space (e.g., adjusting position sizes or initiating trades). The environment (simulated FX market) provides feedback in the form of a reward signal, usually based on the profit or loss incurred from the trade.
Sophisticated RL algorithms, such as Deep Q-Networks (DQNs) or Policy Gradient methods (e.g., Proximal Policy Optimization - PPO), are often employed. These algorithms use neural networks to approximate the optimal policy (mapping states to actions) or value function (estimating the expected future reward for a given state-action pair). The agent learns through exploration (trying out different actions) and exploitation (leveraging actions that have yielded high rewards in the past).
One of the potential advantages of using RL agents for FX prediction is their ability to learn complex, non-linear relationships and adapt to changing market conditions without explicit human intervention. They can also potentially identify subtle patterns and trading opportunities that might be missed by traditional analytical methods. However, training RL agents for FX trading can be challenging. It requires large amounts of realistic market data for effective learning, and the design of the reward function and state space is crucial for the agent's performance. Overfitting to the training data and ensuring the agent generalizes well to unseen market conditions are also significant hurdles. Furthermore, the inherent stochasticity and high noise levels in the FX market can make it difficult for RL agents to consistently generate profitable predictions. Despite these challenges, the field of applying RL to algorithmic trading, including Forex, is an active area of research and development.
Like 0
lee9037
Trader
Hot content
Industry
Event-A comment a day,Keep rewards worthy up to$27
Industry
Nigeria Event Giveaway-Win₦5000 Mobilephone Credit
Industry
Nigeria Event Giveaway-Win ₦2500 MobilePhoneCredit
Industry
South Africa Event-Come&Win 240ZAR Phone Credit
Industry
Nigeria Event-Discuss Forex&Win2500NGN PhoneCredit
Industry
[Nigeria Event]Discuss&win 2500 Naira Phone Credit
Forum category
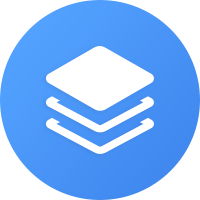
Platform
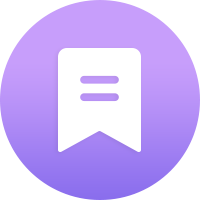
Exhibition
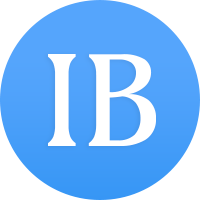
Agent
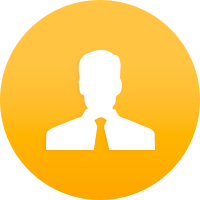
Recruitment
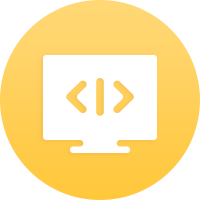
EA
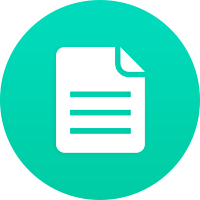
Industry
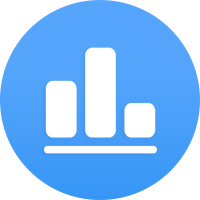
Market
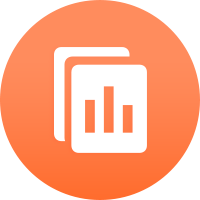
Index
Predicting forex pairs withreinforcement learning
#CurrencyPairPrediction
Predicting Forex (FX) pair movements using reinforcement learning (RL) agents involves training autonomous agents to learn optimal trading strategies through trial and error in a simulated FX market environment. These agents interact with the market, make trading decisions (buy, sell, or hold), and receive rewards (positive or negative) based on the profitability of their actions. Over time, the RL agent learns to maximize its cumulative reward, effectively discovering profitable trading strategies without explicit programming of specific rules.
The process typically involves defining a state space that includes relevant market data, such as historical price action, technical indicators (e.g., moving averages, RSI, MACD), order book information, and potentially even sentiment data. The agent then takes actions within an action space (e.g., adjusting position sizes or initiating trades). The environment (simulated FX market) provides feedback in the form of a reward signal, usually based on the profit or loss incurred from the trade.
Sophisticated RL algorithms, such as Deep Q-Networks (DQNs) or Policy Gradient methods (e.g., Proximal Policy Optimization - PPO), are often employed. These algorithms use neural networks to approximate the optimal policy (mapping states to actions) or value function (estimating the expected future reward for a given state-action pair). The agent learns through exploration (trying out different actions) and exploitation (leveraging actions that have yielded high rewards in the past).
One of the potential advantages of using RL agents for FX prediction is their ability to learn complex, non-linear relationships and adapt to changing market conditions without explicit human intervention. They can also potentially identify subtle patterns and trading opportunities that might be missed by traditional analytical methods. However, training RL agents for FX trading can be challenging. It requires large amounts of realistic market data for effective learning, and the design of the reward function and state space is crucial for the agent's performance. Overfitting to the training data and ensuring the agent generalizes well to unseen market conditions are also significant hurdles. Furthermore, the inherent stochasticity and high noise levels in the FX market can make it difficult for RL agents to consistently generate profitable predictions. Despite these challenges, the field of applying RL to algorithmic trading, including Forex, is an active area of research and development.
Like 0
I want to comment, too
Submit
0Comments
There is no comment yet. Make the first one.
Submit
There is no comment yet. Make the first one.