Malaysia
2025-04-28 11:15
IndustryMulti-head attention models forforex prediction
#CurrencyPairPrediction
Multi-head attention models represent a significant advancement in deep learning for time series forecasting, including the complex task of Forex (FX) prediction. These models, inspired by the Transformer architecture initially developed for natural language processing, excel at capturing intricate dependencies within sequential data by allowing the model to attend to different parts of the input sequence with varying degrees of importance.
The core idea behind multi-head attention is to use multiple "attention heads" in parallel. Each head learns different weightings of the input features, enabling the model to capture a wider range of relationships within the historical price data and other relevant inputs, such as technical indicators or even sentiment data. By focusing on different aspects of the input simultaneously, the model can better understand the complex interplay of factors that drive currency movements.
Compared to traditional Recurrent Neural Networks (RNNs) like LSTMs or GRUs, multi-head attention mechanisms can handle longer sequences more effectively and in parallel, potentially leading to faster training times and the ability to capture long-range dependencies that might be missed by recurrent architectures. Moreover, the attention weights learned by the model can offer a degree of interpretability, showing which historical data points or features the model deemed most important for its predictions at a given time.
In the context of FX prediction, multi-head attention models can analyze patterns across different timeframes and identify crucial relationships between various currency pairs or economic indicators. For instance, the model might learn to pay more attention to specific historical price patterns, volatility spikes, or the release of high-impact economic news when predicting the future direction of a currency pair.
However, like other deep learning models, multi-head attention networks require substantial amounts of high-quality data for training and can be computationally intensive. Overfitting to the training data is also a risk that needs to be carefully managed through techniques like regularization and cross-validation. Despite these challenges, the ability of multi-head attention models to capture complex, non-linear relationships and long-term dependencies makes them a promising tool for improving the accuracy and robustness of Forex forecasting. Hybrid models combining multi-head attention with other deep learning architectures or traditional time series analysis techniques are also being explored to further enhance predictive performance.
Like 0
lov920
Trader
Hot content
Industry
Event-A comment a day,Keep rewards worthy up to$27
Industry
Nigeria Event Giveaway-Win₦5000 Mobilephone Credit
Industry
Nigeria Event Giveaway-Win ₦2500 MobilePhoneCredit
Industry
South Africa Event-Come&Win 240ZAR Phone Credit
Industry
Nigeria Event-Discuss Forex&Win2500NGN PhoneCredit
Industry
[Nigeria Event]Discuss&win 2500 Naira Phone Credit
Forum category
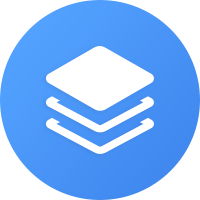
Platform
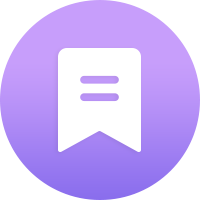
Exhibition
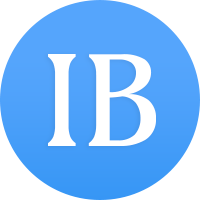
Agent
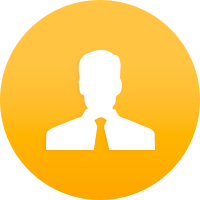
Recruitment
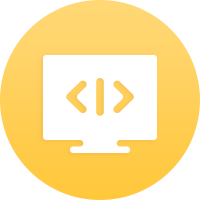
EA
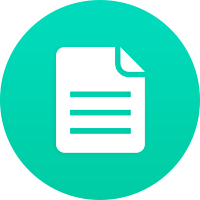
Industry
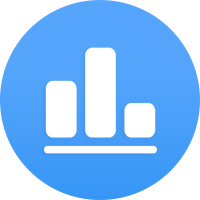
Market
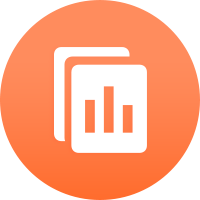
Index
Multi-head attention models forforex prediction
#CurrencyPairPrediction
Multi-head attention models represent a significant advancement in deep learning for time series forecasting, including the complex task of Forex (FX) prediction. These models, inspired by the Transformer architecture initially developed for natural language processing, excel at capturing intricate dependencies within sequential data by allowing the model to attend to different parts of the input sequence with varying degrees of importance.
The core idea behind multi-head attention is to use multiple "attention heads" in parallel. Each head learns different weightings of the input features, enabling the model to capture a wider range of relationships within the historical price data and other relevant inputs, such as technical indicators or even sentiment data. By focusing on different aspects of the input simultaneously, the model can better understand the complex interplay of factors that drive currency movements.
Compared to traditional Recurrent Neural Networks (RNNs) like LSTMs or GRUs, multi-head attention mechanisms can handle longer sequences more effectively and in parallel, potentially leading to faster training times and the ability to capture long-range dependencies that might be missed by recurrent architectures. Moreover, the attention weights learned by the model can offer a degree of interpretability, showing which historical data points or features the model deemed most important for its predictions at a given time.
In the context of FX prediction, multi-head attention models can analyze patterns across different timeframes and identify crucial relationships between various currency pairs or economic indicators. For instance, the model might learn to pay more attention to specific historical price patterns, volatility spikes, or the release of high-impact economic news when predicting the future direction of a currency pair.
However, like other deep learning models, multi-head attention networks require substantial amounts of high-quality data for training and can be computationally intensive. Overfitting to the training data is also a risk that needs to be carefully managed through techniques like regularization and cross-validation. Despite these challenges, the ability of multi-head attention models to capture complex, non-linear relationships and long-term dependencies makes them a promising tool for improving the accuracy and robustness of Forex forecasting. Hybrid models combining multi-head attention with other deep learning architectures or traditional time series analysis techniques are also being explored to further enhance predictive performance.
Like 0
I want to comment, too
Submit
0Comments
There is no comment yet. Make the first one.
Submit
There is no comment yet. Make the first one.