Malaysia
2025-04-28 11:08
IndustryComparing traditional models vsdeep learning in FX
#CurrencyPairPrediction
Comparing traditional statistical models and modern deep learning techniques for Forex (FX) prediction reveals distinct advantages and disadvantages for each approach.
Traditional Models: These models, including ARIMA, GARCH, and regression analysis, have been staples in time series forecasting. They are often linear or have a limited capacity to capture non-linear relationships in the data. Traditional models benefit from interpretability, as the impact of input variables on the output is usually clear and quantifiable. They also typically require less computational power and smaller datasets for training. However, their ability to model the complex, dynamic, and often chaotic nature of the FX market is limited, potentially leading to lower accuracy, especially in the presence of high volatility and non-linear patterns.
Deep Learning Models: In contrast, deep learning models, such as Recurrent Neural Networks (RNNs), including LSTMs and GRUs, and Convolutional Neural Networks (CNNs), excel at learning intricate non-linear dependencies and patterns from large datasets. Their ability to automatically extract relevant features from raw data can be a significant advantage in the FX market, which is influenced by numerous interacting factors. Studies suggest that deep learning models can outperform traditional methods by capturing complex nuances in the data, leading to more accurate predictions. However, these models are often considered "black boxes" with limited interpretability. They also demand substantial computational resources and large volumes of data for effective training. Overfitting can also be a significant challenge if not properly addressed.
Hybrid Approaches: Recognizing the strengths of both, some researchers are exploring hybrid models that combine traditional statistical methods with deep learning techniques. These approaches aim to leverage the interpretability of traditional models and the pattern recognition capabilities of deep learning to achieve potentially more robust and insightful FX predictions.
Ultimately, the choice between traditional and deep learning models for FX prediction depends on the specific goals, available data, computational resources, and the desired level of interpretability. While deep learning has shown promise in capturing complex market dynamics, traditional models still offer value, particularly when interpretability and resource efficiency are paramount. The evolving landscape of FX prediction suggests that hybrid approaches may offer a balanced and potentially superior alternative.
Like 0
mgann
Trader
Hot content
Industry
Event-A comment a day,Keep rewards worthy up to$27
Industry
Nigeria Event Giveaway-Win₦5000 Mobilephone Credit
Industry
Nigeria Event Giveaway-Win ₦2500 MobilePhoneCredit
Industry
South Africa Event-Come&Win 240ZAR Phone Credit
Industry
Nigeria Event-Discuss Forex&Win2500NGN PhoneCredit
Industry
[Nigeria Event]Discuss&win 2500 Naira Phone Credit
Forum category
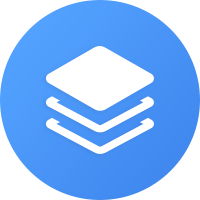
Platform
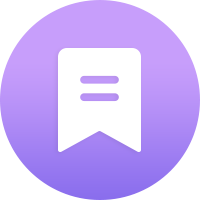
Exhibition
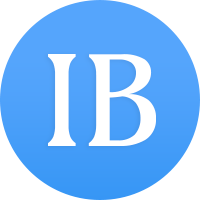
Agent
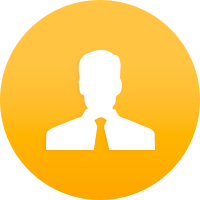
Recruitment
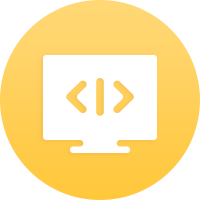
EA
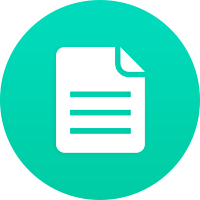
Industry
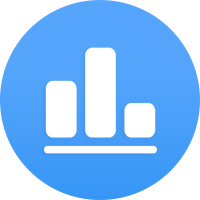
Market
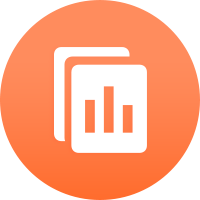
Index
Comparing traditional models vsdeep learning in FX
#CurrencyPairPrediction
Comparing traditional statistical models and modern deep learning techniques for Forex (FX) prediction reveals distinct advantages and disadvantages for each approach.
Traditional Models: These models, including ARIMA, GARCH, and regression analysis, have been staples in time series forecasting. They are often linear or have a limited capacity to capture non-linear relationships in the data. Traditional models benefit from interpretability, as the impact of input variables on the output is usually clear and quantifiable. They also typically require less computational power and smaller datasets for training. However, their ability to model the complex, dynamic, and often chaotic nature of the FX market is limited, potentially leading to lower accuracy, especially in the presence of high volatility and non-linear patterns.
Deep Learning Models: In contrast, deep learning models, such as Recurrent Neural Networks (RNNs), including LSTMs and GRUs, and Convolutional Neural Networks (CNNs), excel at learning intricate non-linear dependencies and patterns from large datasets. Their ability to automatically extract relevant features from raw data can be a significant advantage in the FX market, which is influenced by numerous interacting factors. Studies suggest that deep learning models can outperform traditional methods by capturing complex nuances in the data, leading to more accurate predictions. However, these models are often considered "black boxes" with limited interpretability. They also demand substantial computational resources and large volumes of data for effective training. Overfitting can also be a significant challenge if not properly addressed.
Hybrid Approaches: Recognizing the strengths of both, some researchers are exploring hybrid models that combine traditional statistical methods with deep learning techniques. These approaches aim to leverage the interpretability of traditional models and the pattern recognition capabilities of deep learning to achieve potentially more robust and insightful FX predictions.
Ultimately, the choice between traditional and deep learning models for FX prediction depends on the specific goals, available data, computational resources, and the desired level of interpretability. While deep learning has shown promise in capturing complex market dynamics, traditional models still offer value, particularly when interpretability and resource efficiency are paramount. The evolving landscape of FX prediction suggests that hybrid approaches may offer a balanced and potentially superior alternative.
Like 0
I want to comment, too
Submit
0Comments
There is no comment yet. Make the first one.
Submit
There is no comment yet. Make the first one.