Thailand
2025-04-28 10:15
IndustryEnsemble Methods for Forex Rate Forecasting
#AIImpactOnForex
Sure! Here's a short summary:
Ensemble Methods for Forex Rate Forecasting
Ensemble methods combine multiple models to improve the accuracy and robustness of foreign exchange (forex) rate forecasting. In volatile forex markets, single models often struggle with noise and nonlinearity. Ensemble techniques like Bagging, Boosting, and Stacking help by leveraging the strengths of diverse algorithms.
Bagging (e.g., Random Forests) reduces variance by averaging predictions from many models trained on different data subsets.
Boosting (e.g., XGBoost, AdaBoost) reduces bias by sequentially training models that focus on correcting previous errors.
Stacking blends different model types (like neural networks, SVMs, and decision trees) and uses a meta-model to learn how to best combine them.
By aggregating multiple predictions, ensemble methods typically outperform individual models, providing more stable and accurate forex forecasts.
Like 0
sara967
Trader
Hot content
Industry
Event-A comment a day,Keep rewards worthy up to$27
Industry
Nigeria Event Giveaway-Win₦5000 Mobilephone Credit
Industry
Nigeria Event Giveaway-Win ₦2500 MobilePhoneCredit
Industry
South Africa Event-Come&Win 240ZAR Phone Credit
Industry
Nigeria Event-Discuss Forex&Win2500NGN PhoneCredit
Industry
[Nigeria Event]Discuss&win 2500 Naira Phone Credit
Forum category
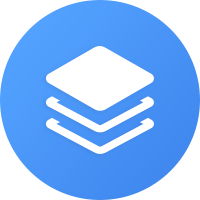
Platform
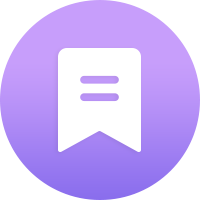
Exhibition
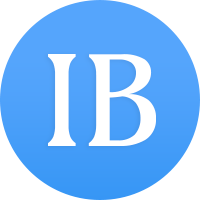
Agent
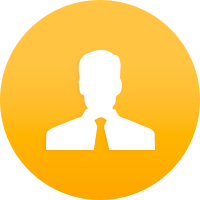
Recruitment
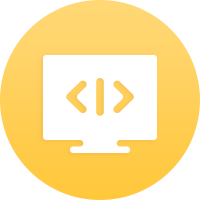
EA
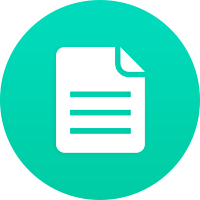
Industry
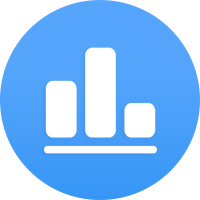
Market
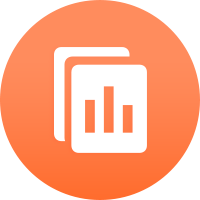
Index
Ensemble Methods for Forex Rate Forecasting
#AIImpactOnForex
Sure! Here's a short summary:
Ensemble Methods for Forex Rate Forecasting
Ensemble methods combine multiple models to improve the accuracy and robustness of foreign exchange (forex) rate forecasting. In volatile forex markets, single models often struggle with noise and nonlinearity. Ensemble techniques like Bagging, Boosting, and Stacking help by leveraging the strengths of diverse algorithms.
Bagging (e.g., Random Forests) reduces variance by averaging predictions from many models trained on different data subsets.
Boosting (e.g., XGBoost, AdaBoost) reduces bias by sequentially training models that focus on correcting previous errors.
Stacking blends different model types (like neural networks, SVMs, and decision trees) and uses a meta-model to learn how to best combine them.
By aggregating multiple predictions, ensemble methods typically outperform individual models, providing more stable and accurate forex forecasts.
Like 0
I want to comment, too
Submit
0Comments
There is no comment yet. Make the first one.
Submit
There is no comment yet. Make the first one.