Thailand
2025-04-28 10:12
IndustrySupport Vector Machines in Currency Prediction
#AIImpactOnForex
Support Vector Machines (SVM) in Currency Prediction
Support Vector Machines (SVM) are powerful supervised learning models used for classification and regression tasks. In currency prediction, SVMs help forecast future exchange rates by identifying patterns in historical financial data. They work by finding the optimal hyperplane that separates data points of different classes (e.g., currency uptrend vs. downtrend) with maximum margin. SVMs are effective in handling non-linear relationships by using kernel functions, such as the radial basis function (RBF). Their strength lies in their ability to generalize well even with limited or noisy data, making them popular for financial time series analysis. However, SVM performance heavily depends on proper feature selection, parameter tuning, and data preprocessing.
Like 0
kim6441
Trader
Hot content
Industry
Event-A comment a day,Keep rewards worthy up to$27
Industry
Nigeria Event Giveaway-Win₦5000 Mobilephone Credit
Industry
Nigeria Event Giveaway-Win ₦2500 MobilePhoneCredit
Industry
South Africa Event-Come&Win 240ZAR Phone Credit
Industry
Nigeria Event-Discuss Forex&Win2500NGN PhoneCredit
Industry
[Nigeria Event]Discuss&win 2500 Naira Phone Credit
Forum category
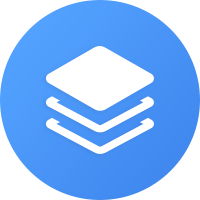
Platform
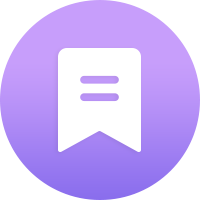
Exhibition
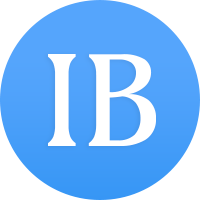
Agent
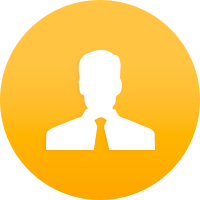
Recruitment
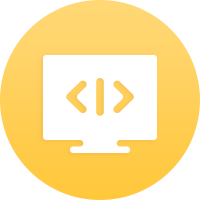
EA
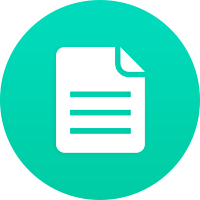
Industry
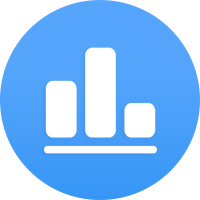
Market
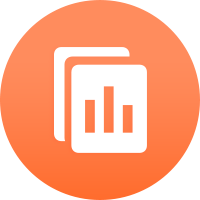
Index
Support Vector Machines in Currency Prediction
#AIImpactOnForex
Support Vector Machines (SVM) in Currency Prediction
Support Vector Machines (SVM) are powerful supervised learning models used for classification and regression tasks. In currency prediction, SVMs help forecast future exchange rates by identifying patterns in historical financial data. They work by finding the optimal hyperplane that separates data points of different classes (e.g., currency uptrend vs. downtrend) with maximum margin. SVMs are effective in handling non-linear relationships by using kernel functions, such as the radial basis function (RBF). Their strength lies in their ability to generalize well even with limited or noisy data, making them popular for financial time series analysis. However, SVM performance heavily depends on proper feature selection, parameter tuning, and data preprocessing.
Like 0
I want to comment, too
Submit
0Comments
There is no comment yet. Make the first one.
Submit
There is no comment yet. Make the first one.