Malaysia
2025-04-25 17:24
IndustryDetecting currrency shifts with supervised models
#CurrencyPairPrediction
Detecting Currency Shifts with Supervised Models:
Detecting currency shifts with supervised models involves training algorithms to identify significant changes or trends in currency values. Key components include:
1. Labeling Shifts: Define currency shifts (e.g., upward/downward movements or volatility spikes) using thresholds or domain rules to create target labels.
2. Feature Engineering: Use indicators like exchange rates, technical indicators (e.g., moving averages, RSI), macroeconomic data, and lagged features.
3. Model Choices: Tree-based models (e.g., Random Forest, XGBoost), neural networks, or logistic regression can classify or predict shifts.
4. Performance Metrics: Use accuracy, precision-recall, or F1-score to evaluate how well the model detects true shifts versus noise.
These models help in early detection of market trends, enabling timely trading or hedging decisions.
Like 0
Sihan
Trader
Hot content
Industry
Event-A comment a day,Keep rewards worthy up to$27
Industry
Nigeria Event Giveaway-Win₦5000 Mobilephone Credit
Industry
Nigeria Event Giveaway-Win ₦2500 MobilePhoneCredit
Industry
South Africa Event-Come&Win 240ZAR Phone Credit
Industry
Nigeria Event-Discuss Forex&Win2500NGN PhoneCredit
Industry
[Nigeria Event]Discuss&win 2500 Naira Phone Credit
Forum category
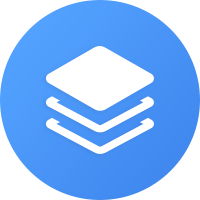
Platform
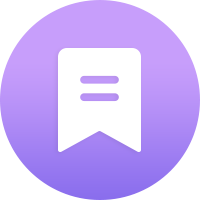
Exhibition
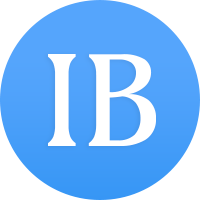
Agent
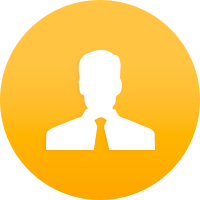
Recruitment
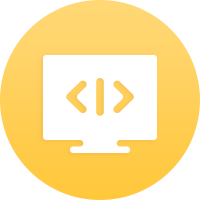
EA
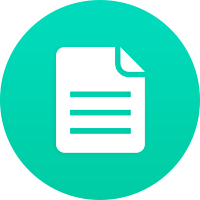
Industry
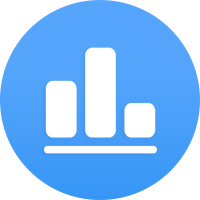
Market
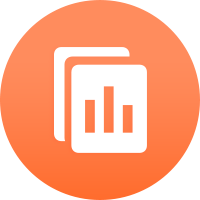
Index
Detecting currrency shifts with supervised models
#CurrencyPairPrediction
Detecting Currency Shifts with Supervised Models:
Detecting currency shifts with supervised models involves training algorithms to identify significant changes or trends in currency values. Key components include:
1. Labeling Shifts: Define currency shifts (e.g., upward/downward movements or volatility spikes) using thresholds or domain rules to create target labels.
2. Feature Engineering: Use indicators like exchange rates, technical indicators (e.g., moving averages, RSI), macroeconomic data, and lagged features.
3. Model Choices: Tree-based models (e.g., Random Forest, XGBoost), neural networks, or logistic regression can classify or predict shifts.
4. Performance Metrics: Use accuracy, precision-recall, or F1-score to evaluate how well the model detects true shifts versus noise.
These models help in early detection of market trends, enabling timely trading or hedging decisions.
Like 0
I want to comment, too
Submit
0Comments
There is no comment yet. Make the first one.
Submit
There is no comment yet. Make the first one.