Malaysia
2025-04-25 17:18
IndustryData normalization techniques for currency feature
#CurrencyPairPrediction
Data Normalization Techniques for Currency Features:
Data normalization for currency features involves scaling monetary values to ensure consistency and improve model performance. Common techniques include:
1. Min-Max Scaling: Transforms currency values to a fixed range, typically [0, 1], helping models treat different currencies uniformly.
2. Z-score Standardization: Centers currency data by subtracting the mean and dividing by standard deviation, making it suitable for algorithms sensitive to feature variance.
3. Log Transformation: Reduces skewness in highly variable currency data, making distributions more normal-like.
4. Currency Conversion: Converts all values to a common base currency using exchange rates, ensuring comparability across international data.
5. Robust Scaling: Uses median and interquartile range to scale data, effective for currency features with outliers.
These techniques help in improving model accuracy, training stability, and comparability across diverse datasets.
Like 0
Messi3926
Trader
Hot content
Industry
Event-A comment a day,Keep rewards worthy up to$27
Industry
Nigeria Event Giveaway-Win₦5000 Mobilephone Credit
Industry
Nigeria Event Giveaway-Win ₦2500 MobilePhoneCredit
Industry
South Africa Event-Come&Win 240ZAR Phone Credit
Industry
Nigeria Event-Discuss Forex&Win2500NGN PhoneCredit
Industry
[Nigeria Event]Discuss&win 2500 Naira Phone Credit
Forum category
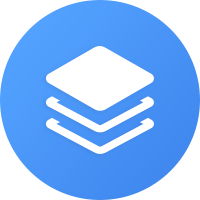
Platform
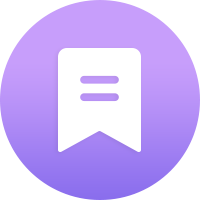
Exhibition
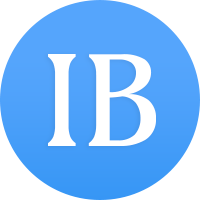
Agent
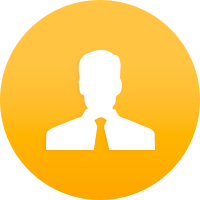
Recruitment
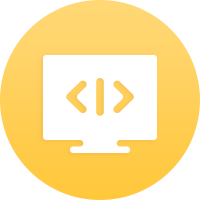
EA
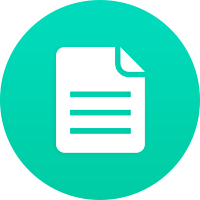
Industry
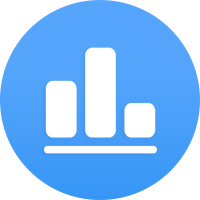
Market
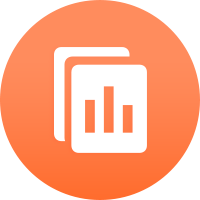
Index
Data normalization techniques for currency feature
#CurrencyPairPrediction
Data Normalization Techniques for Currency Features:
Data normalization for currency features involves scaling monetary values to ensure consistency and improve model performance. Common techniques include:
1. Min-Max Scaling: Transforms currency values to a fixed range, typically [0, 1], helping models treat different currencies uniformly.
2. Z-score Standardization: Centers currency data by subtracting the mean and dividing by standard deviation, making it suitable for algorithms sensitive to feature variance.
3. Log Transformation: Reduces skewness in highly variable currency data, making distributions more normal-like.
4. Currency Conversion: Converts all values to a common base currency using exchange rates, ensuring comparability across international data.
5. Robust Scaling: Uses median and interquartile range to scale data, effective for currency features with outliers.
These techniques help in improving model accuracy, training stability, and comparability across diverse datasets.
Like 0
I want to comment, too
Submit
0Comments
There is no comment yet. Make the first one.
Submit
There is no comment yet. Make the first one.