Malaysia
2025-04-25 16:58
IndustryUsing SHAP values to explain model decisions
#CurrencyPairPrediction
Using SHAP Values to Explain Model Decisions
SHAP (SHapley Additive exPlanations) values provide a powerful method to explain the output of machine learning models by quantifying each feature's contribution to a specific prediction:
Feature Attribution: SHAP shows how much each input feature pushed a prediction higher or lower, helping users understand model reasoning.
Consistent and Local Explanations: It offers explanations for individual predictions (local) while maintaining fairness and consistency across the dataset (global).
Model-Agnostic: SHAP can be applied to any machine learning model, including complex ones like gradient boosting and neural networks.
Improved Trust and Transparency: In finance, SHAP helps traders, analysts, and regulators interpret decisions, improving trust in AI-driven strategies.
SHAP enhances model interpretability by making predictions more understandable, auditable, and actionable.
Like 0
Uzack
Trader
Hot content
Industry
Event-A comment a day,Keep rewards worthy up to$27
Industry
Nigeria Event Giveaway-Win₦5000 Mobilephone Credit
Industry
Nigeria Event Giveaway-Win ₦2500 MobilePhoneCredit
Industry
South Africa Event-Come&Win 240ZAR Phone Credit
Industry
Nigeria Event-Discuss Forex&Win2500NGN PhoneCredit
Industry
[Nigeria Event]Discuss&win 2500 Naira Phone Credit
Forum category
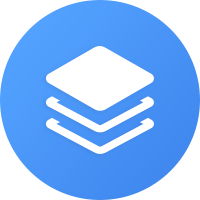
Platform
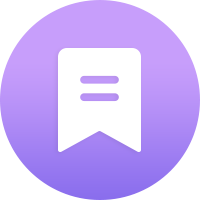
Exhibition
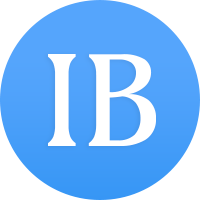
Agent
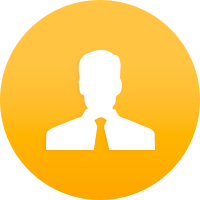
Recruitment
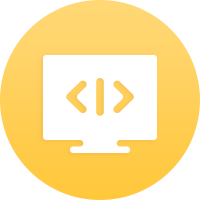
EA
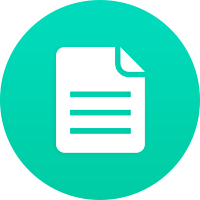
Industry
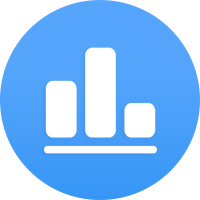
Market
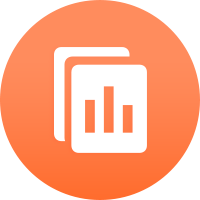
Index
Using SHAP values to explain model decisions
#CurrencyPairPrediction
Using SHAP Values to Explain Model Decisions
SHAP (SHapley Additive exPlanations) values provide a powerful method to explain the output of machine learning models by quantifying each feature's contribution to a specific prediction:
Feature Attribution: SHAP shows how much each input feature pushed a prediction higher or lower, helping users understand model reasoning.
Consistent and Local Explanations: It offers explanations for individual predictions (local) while maintaining fairness and consistency across the dataset (global).
Model-Agnostic: SHAP can be applied to any machine learning model, including complex ones like gradient boosting and neural networks.
Improved Trust and Transparency: In finance, SHAP helps traders, analysts, and regulators interpret decisions, improving trust in AI-driven strategies.
SHAP enhances model interpretability by making predictions more understandable, auditable, and actionable.
Like 0
I want to comment, too
Submit
0Comments
There is no comment yet. Make the first one.
Submit
There is no comment yet. Make the first one.