Malaysia
2025-04-25 16:49
IndustryIntegrating supervised learning in trading bots
#CurrencyPairPrediction
Integrating Supervised Learning in Trading Bots
Integrating supervised learning into trading bots enhances decision-making by allowing the bot to predict market movements based on historical data. Key components include:
Model Integration: The trained model is embedded into the bot’s architecture to generate buy/sell signals based on real-time inputs.
Feature Engineering: Relevant financial indicators, price patterns, and news sentiment are used as inputs to improve prediction accuracy.
Execution Logic: The bot must translate model predictions into actionable trades while considering timing, slippage, and transaction costs.
Risk Management: Stop-loss, position sizing, and capital allocation strategies are essential to control risk.
Continuous Learning: Models can be periodically retrained or updated to adapt to changing market conditions.
This integration enables automated, data-driven trading with the potential for improved consistency and reduced emotional bias.
Like 0
Donda915
Trader
Hot content
Industry
Event-A comment a day,Keep rewards worthy up to$27
Industry
Nigeria Event Giveaway-Win₦5000 Mobilephone Credit
Industry
Nigeria Event Giveaway-Win ₦2500 MobilePhoneCredit
Industry
South Africa Event-Come&Win 240ZAR Phone Credit
Industry
Nigeria Event-Discuss Forex&Win2500NGN PhoneCredit
Industry
[Nigeria Event]Discuss&win 2500 Naira Phone Credit
Forum category
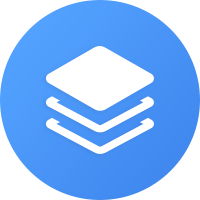
Platform
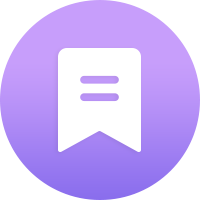
Exhibition
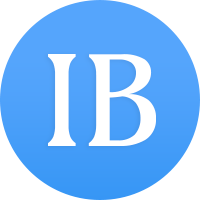
Agent
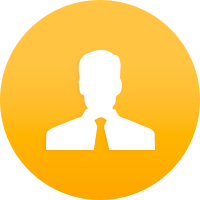
Recruitment
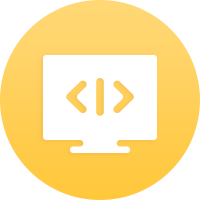
EA
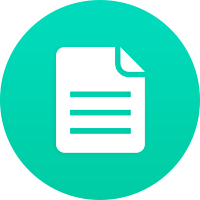
Industry
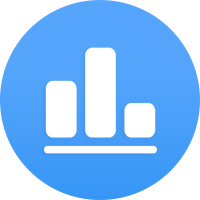
Market
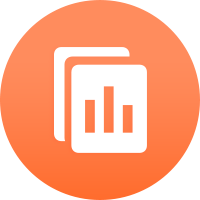
Index
Integrating supervised learning in trading bots
#CurrencyPairPrediction
Integrating Supervised Learning in Trading Bots
Integrating supervised learning into trading bots enhances decision-making by allowing the bot to predict market movements based on historical data. Key components include:
Model Integration: The trained model is embedded into the bot’s architecture to generate buy/sell signals based on real-time inputs.
Feature Engineering: Relevant financial indicators, price patterns, and news sentiment are used as inputs to improve prediction accuracy.
Execution Logic: The bot must translate model predictions into actionable trades while considering timing, slippage, and transaction costs.
Risk Management: Stop-loss, position sizing, and capital allocation strategies are essential to control risk.
Continuous Learning: Models can be periodically retrained or updated to adapt to changing market conditions.
This integration enables automated, data-driven trading with the potential for improved consistency and reduced emotional bias.
Like 0
I want to comment, too
Submit
0Comments
There is no comment yet. Make the first one.
Submit
There is no comment yet. Make the first one.