Malaysia
2025-04-25 16:35
IndustryHigh-frequency data and supervised learning challe
#CurrencyPairPrediction
High-Frequency Data and Supervised Learning Challenges
High-frequency data (HFD), often encountered in finance and IoT applications, refers to data collected at very short intervals—sometimes milliseconds or microseconds apart. While this provides detailed insights, it also introduces several challenges for supervised learning models:
1. Volume and Velocity: The massive volume and speed of data require high computational resources and efficient algorithms.
2. Noise and Sparsity: HFD is often noisy, with irregular patterns and outliers, making it harder for models to learn meaningful patterns.
3. Labeling Difficulty: Obtaining accurate, timely labels at high frequency is challenging, leading to weak supervision or delayed feedback.
4. Overfitting Risk: The dense, redundant nature of HFD can cause models to overfit, especially without proper regularization.
5. Concept Drift: Rapid changes in underlying data distributions over time necessitate frequent model retraining or online learning strategies.
These factors make supervised learning on HFD complex, demanding robust preprocessing, model tuning, and adaptive learning techniques.
Like 0
simao5427
Trader
Hot content
Industry
Event-A comment a day,Keep rewards worthy up to$27
Industry
Nigeria Event Giveaway-Win₦5000 Mobilephone Credit
Industry
Nigeria Event Giveaway-Win ₦2500 MobilePhoneCredit
Industry
South Africa Event-Come&Win 240ZAR Phone Credit
Industry
Nigeria Event-Discuss Forex&Win2500NGN PhoneCredit
Industry
[Nigeria Event]Discuss&win 2500 Naira Phone Credit
Forum category
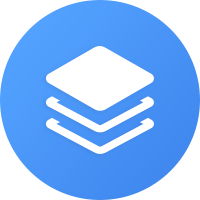
Platform
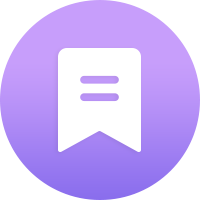
Exhibition
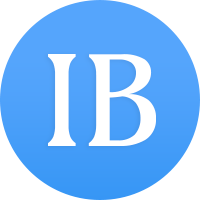
Agent
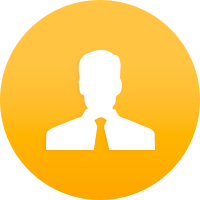
Recruitment
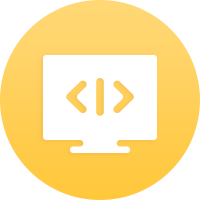
EA
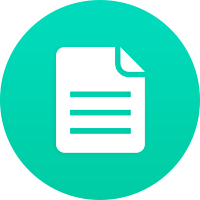
Industry
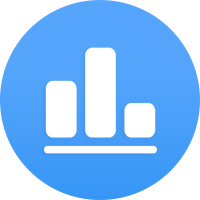
Market
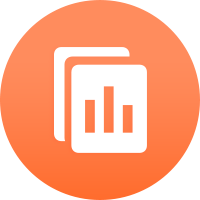
Index
High-frequency data and supervised learning challe
#CurrencyPairPrediction
High-Frequency Data and Supervised Learning Challenges
High-frequency data (HFD), often encountered in finance and IoT applications, refers to data collected at very short intervals—sometimes milliseconds or microseconds apart. While this provides detailed insights, it also introduces several challenges for supervised learning models:
1. Volume and Velocity: The massive volume and speed of data require high computational resources and efficient algorithms.
2. Noise and Sparsity: HFD is often noisy, with irregular patterns and outliers, making it harder for models to learn meaningful patterns.
3. Labeling Difficulty: Obtaining accurate, timely labels at high frequency is challenging, leading to weak supervision or delayed feedback.
4. Overfitting Risk: The dense, redundant nature of HFD can cause models to overfit, especially without proper regularization.
5. Concept Drift: Rapid changes in underlying data distributions over time necessitate frequent model retraining or online learning strategies.
These factors make supervised learning on HFD complex, demanding robust preprocessing, model tuning, and adaptive learning techniques.
Like 0
I want to comment, too
Submit
0Comments
There is no comment yet. Make the first one.
Submit
There is no comment yet. Make the first one.