Thailand
2025-04-25 13:10
IndustryDeveloping Al-based countertrendstrategies
#AIImpactOnForex
Developing AI-based countertrend strategies in forex involves creating intelligent systems that can identify and capitalize on potential reversals against the prevailing trend. This requires AI to go beyond simple pattern recognition and understand the nuances of trend exhaustion and reversal signals.
Identifying Potential Reversal Zones: AI algorithms can be trained on vast historical datasets to recognize conditions where trends are likely to weaken and reverse. This includes analyzing price action patterns (e.g., divergences, exhaustion gaps, specific candlestick formations), momentum indicators (e.g., RSI, Stochastic showing overbought/oversold conditions), volume patterns (e.g., decreasing volume during a trend), and even sentiment indicators. Deep learning models, particularly RNNs and CNNs, can excel at identifying these complex, multi-dimensional reversal setups.
Predicting Reversal Strength and Duration: Beyond identifying potential reversals, AI can attempt to predict the strength and duration of the countertrend move. This involves analyzing factors like the steepness of the preceding trend, the confluence of reversal signals, and potential fundamental catalysts. Machine learning models can learn to associate specific conditions with different magnitudes and durations of countertrend movements.
Optimized Entry and Exit Points: AI can use probabilistic models to determine optimal entry points within identified reversal zones, maximizing the risk-reward ratio. It can also dynamically adjust stop-loss levels based on the predicted volatility and the strength of the reversal signal. Similarly, AI can identify optimal exit points based on profit targets, weakening countertrend momentum, or the re-establishment of the original trend.
Adaptive Strategy Parameters: AI-powered countertrend strategies can continuously adapt their parameters based on evolving market conditions. For example, the sensitivity of reversal signals or the aggressiveness of take-profit targets might be adjusted based on overall market volatility or the historical success rate of countertrend trades in the current market regime. Reinforcement learning techniques could even be employed to allow the AI agent to learn and refine its countertrend strategy through direct interaction with the market.
Risk Management: AI plays a crucial role in managing the inherent risks associated with countertrend trading. It can assess the probability of a failed reversal and adjust position sizes accordingly. AI can also implement rules to avoid entering countertrend trades during periods of very strong trending momentum or high-impact news events that could negate reversal signals.
However, developing successful AI-based countertrend strategies requires careful consideration of the higher probability of failure compared to trend-following strategies and the need for robust risk management protocols. The AI must learn to differentiate between minor pullbacks and genuine trend reversals.
Like 0
Lumpur
Trader
Hot content
Industry
Event-A comment a day,Keep rewards worthy up to$27
Industry
Nigeria Event Giveaway-Win₦5000 Mobilephone Credit
Industry
Nigeria Event Giveaway-Win ₦2500 MobilePhoneCredit
Industry
South Africa Event-Come&Win 240ZAR Phone Credit
Industry
Nigeria Event-Discuss Forex&Win2500NGN PhoneCredit
Industry
[Nigeria Event]Discuss&win 2500 Naira Phone Credit
Forum category
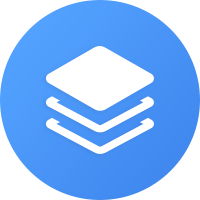
Platform
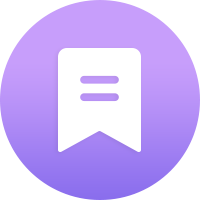
Exhibition
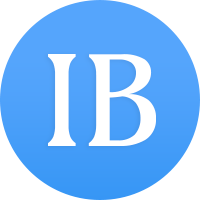
Agent
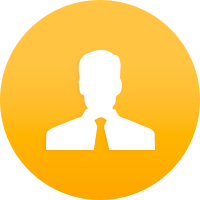
Recruitment
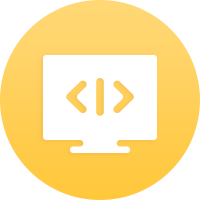
EA
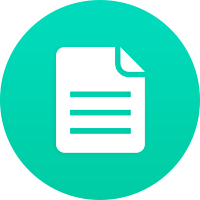
Industry
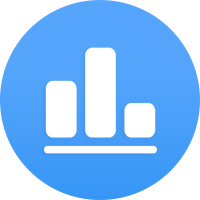
Market
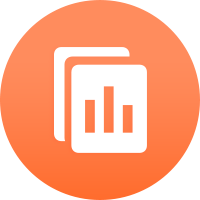
Index
Developing Al-based countertrendstrategies
#AIImpactOnForex
Developing AI-based countertrend strategies in forex involves creating intelligent systems that can identify and capitalize on potential reversals against the prevailing trend. This requires AI to go beyond simple pattern recognition and understand the nuances of trend exhaustion and reversal signals.
Identifying Potential Reversal Zones: AI algorithms can be trained on vast historical datasets to recognize conditions where trends are likely to weaken and reverse. This includes analyzing price action patterns (e.g., divergences, exhaustion gaps, specific candlestick formations), momentum indicators (e.g., RSI, Stochastic showing overbought/oversold conditions), volume patterns (e.g., decreasing volume during a trend), and even sentiment indicators. Deep learning models, particularly RNNs and CNNs, can excel at identifying these complex, multi-dimensional reversal setups.
Predicting Reversal Strength and Duration: Beyond identifying potential reversals, AI can attempt to predict the strength and duration of the countertrend move. This involves analyzing factors like the steepness of the preceding trend, the confluence of reversal signals, and potential fundamental catalysts. Machine learning models can learn to associate specific conditions with different magnitudes and durations of countertrend movements.
Optimized Entry and Exit Points: AI can use probabilistic models to determine optimal entry points within identified reversal zones, maximizing the risk-reward ratio. It can also dynamically adjust stop-loss levels based on the predicted volatility and the strength of the reversal signal. Similarly, AI can identify optimal exit points based on profit targets, weakening countertrend momentum, or the re-establishment of the original trend.
Adaptive Strategy Parameters: AI-powered countertrend strategies can continuously adapt their parameters based on evolving market conditions. For example, the sensitivity of reversal signals or the aggressiveness of take-profit targets might be adjusted based on overall market volatility or the historical success rate of countertrend trades in the current market regime. Reinforcement learning techniques could even be employed to allow the AI agent to learn and refine its countertrend strategy through direct interaction with the market.
Risk Management: AI plays a crucial role in managing the inherent risks associated with countertrend trading. It can assess the probability of a failed reversal and adjust position sizes accordingly. AI can also implement rules to avoid entering countertrend trades during periods of very strong trending momentum or high-impact news events that could negate reversal signals.
However, developing successful AI-based countertrend strategies requires careful consideration of the higher probability of failure compared to trend-following strategies and the need for robust risk management protocols. The AI must learn to differentiate between minor pullbacks and genuine trend reversals.
Like 0
I want to comment, too
Submit
0Comments
There is no comment yet. Make the first one.
Submit
There is no comment yet. Make the first one.